Embedded Learning and Optimization for Interaction-aware Model Predictive Control
The goal of this project is to develop embedded optimization and online learning algorithms for interaction-aware Model Predictive Control (MPC) for autonomous navigation in uncertain environments. In this project, an interaction-aware MPC formulation integrated with online learning has been developed. The integration of online learning in the MPC formulation allows for the customization of the prediction model, tailoring it to the specific dynamics of the system in interaction. This framework serves as the backbone for the further development of embedded learning and optimization methods. In particular, a learning algorithm is developed to identify parameters in a switching system model with state-dependent transition probability. Such a model has been demonstrated to effectively capture nonlinear dynamics. Further research will be undertaken to develop an efficient algorithm to solve the robust control problem in the presence of state-dependent uncertainty by utilizing the problem structure.
Interaction-aware Model Predictive Control for Autonomous Driving
We propose an interaction-aware stochastic model predictive control (MPC) strategy for lane merging tasks in automated driving. The MPC strategy is integrated with an online learning framework, which models a given driver’s cooperation level as an unknown parameter in a state-dependent probability distribution. The online learning framework adaptively estimates the surrounding vehicle’s cooperation level with the vehicle’s past state trajectory and combines this with a kinematic vehicle model to predict the distribution of a multimodal future state trajectory. Learning is conducted using logistic regression, enabling fast online computations. The multi-future prediction is used in the MPC algorithm to compute the optimal control input while satisfying safety constraints. We demonstrate our algorithm in an interactive lane changing scenario with drivers in different randomly selected cooperation levels.
Read more here
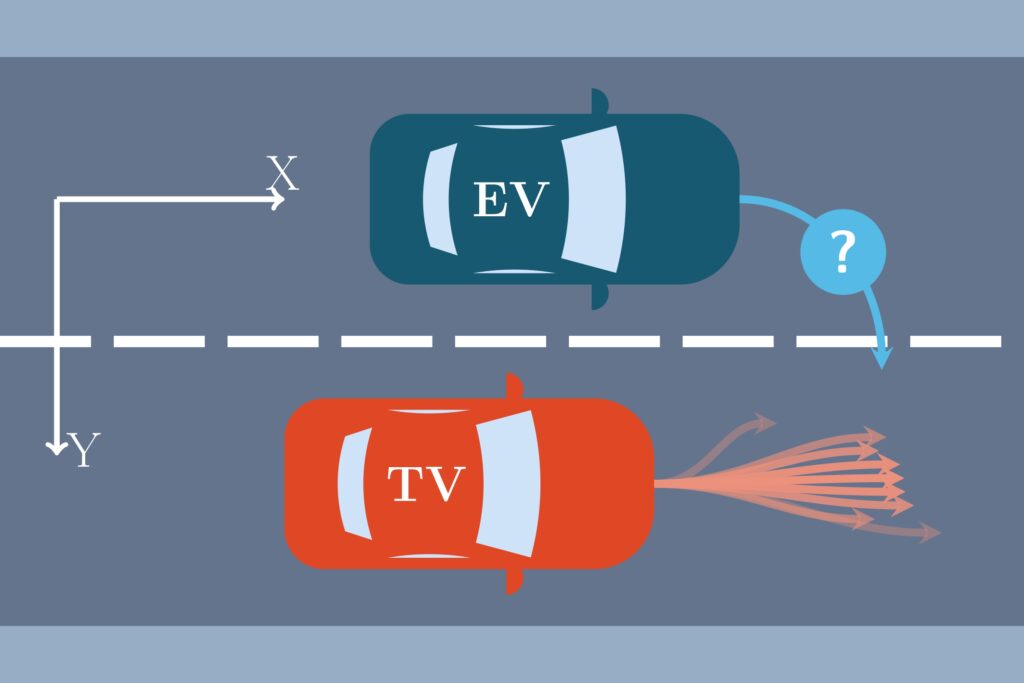
Learning Switching Systems with Presence of State-dependent Distribution
Obtaining a realistic and computationally efficient model will significantly enhance the performance of a model predictive controller. This is especially true for complex scenarios where the system being controlled must interact with other systems. This work aims to construct such a model for controlling a stochastic system with the presence of state-dependent distribution. More specifically, the stochastic system is modeled as a switching system with state-dependent switch probabilities. The parameter of both the switching probability and the sub-systems within the switching model will be identified using data. We demonstrate that the proposed method tackles the nonconvex optimization problem through an iterative approach. At each iteration, the problem is transformed into convex optimization problems that can be solved in parallel.
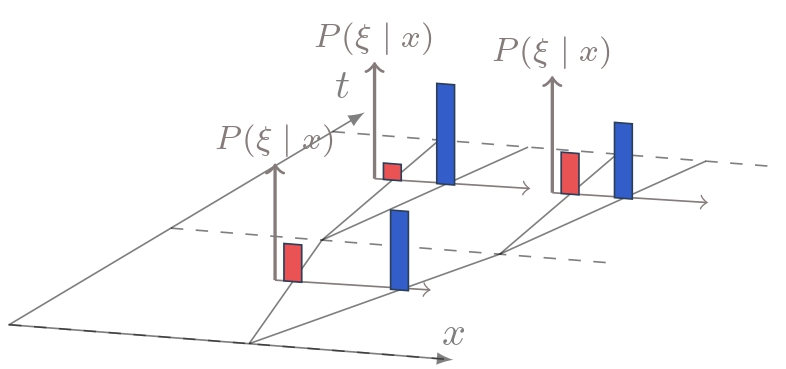
Robust Control Formulation for Switching Systems with State-dependent Distribution
Coming soon…