PhD Candidate in Engineering Science
Department of Electrical Engineering (ESAT), STADIUS division
KU Leuven
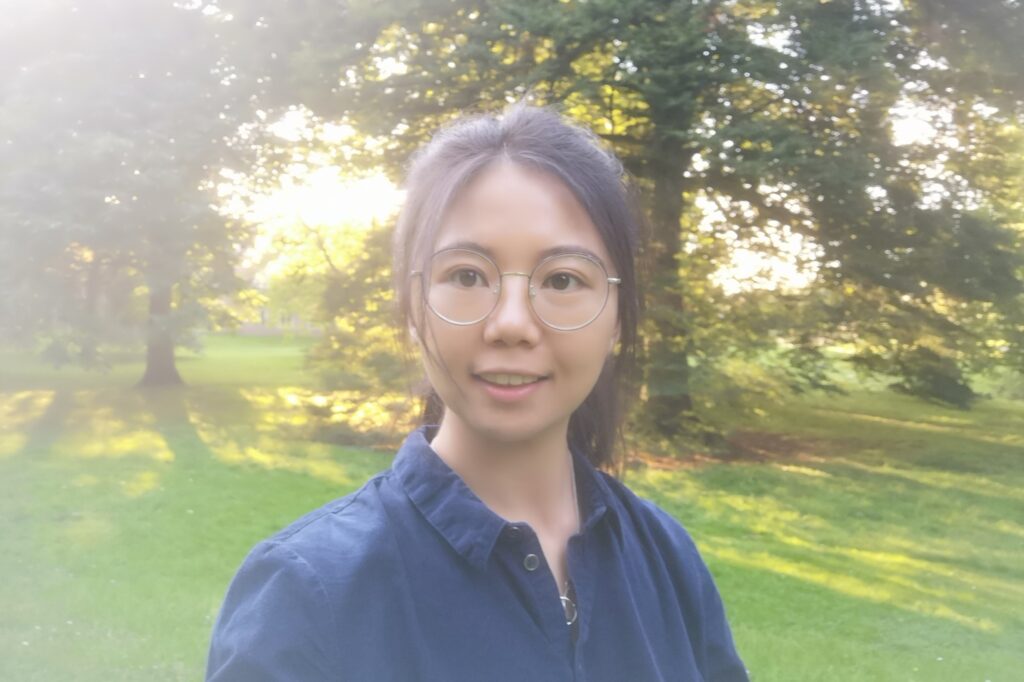
Renzi Wang received her bachelor’s degree in mechatronics with a focus on robotics from Harbin Institute of Technology, China, in 2018, and her master’s degree in mechatronics with a focus on control theory from Karlsruhe Institute of Technology, Germany, in 2021. During her master’s study, she took one exchange semester at EPFL, Switzerland, and completed her master thesis with a topic on extending a data-enabled predictive control method on nonlinear systems there.
Project description
Autonomous navigation in uncertain environments, where vehicles (autonomous cars, robots,
drones) must interact with other vehicles or other users (e.g., pedestrians) is a highly challenging control task. Thus, the development of real-time MPC strategies that takes into account interaction and uncertainty is highly desirable. Traditional means of handling uncertainty such as robust or stochastic approaches are either too conservative or too risk-prone. Moreover, MPC methodologies typically assume that the uncertain behavior of surrounding users is completely exogenous, thus failing to take interaction into account. To explicitly take into account interactions, Renzi is going to develop stochastic models for the surrounding users whose probabilistic structure depends on the states and actions of other users. The transition probabilities are learned online using machine learning in a moving horizon fashion in order to account for dynamic environments. Furthermore, the proposed methodology will provide a natural mechanism for the real-time learning system to safely balance exploitation and exploration, as the optimal input will be exploratory (that is, associated with more uncertain state transitions) only if the risk is sufficiently low. The resulting NMPC formulations will lead optimal control problems that are more complex and of larger scale than what state-of-the-art embedded solvers can handle. The goal of Renzi’s project is to develop embedded optimization and online learning algorithms for interaction-aware MPC for autonomous navigation in uncertain environments.
Publications
Wang, Renzi; Schuurmans, Mathijs; Patrinos, Panagiotis
Risk-Sensitive Model Predictive Control for Interaction-Aware Planning--A Sequential Convexification Algorithm Working paper
2025.
@workingpaper{wang2025risk,
title = {Risk-Sensitive Model Predictive Control for Interaction-Aware Planning--A Sequential Convexification Algorithm},
author = {Renzi Wang and Mathijs Schuurmans and Panagiotis Patrinos},
url = {https://doi.org/10.48550/arXiv.2503.14328},
year = {2025},
date = {2025-03-18},
abstract = {This paper considers risk-sensitive model predictive control for stochastic systems with a decision-dependent distribution. This class of systems is commonly found in human-robot interaction scenarios. We derive computationally tractable convex upper bounds to both the objective function, and to frequently used penalty terms for collision avoidance, allowing us to efficiently solve the generally nonconvex optimal control problem as a sequence of convex problems. Simulations of a robot navigating a corridor demonstrate the effectiveness and the computational advantage of the proposed approach.},
keywords = {},
pubstate = {published},
tppubtype = {workingpaper}
}
Wang, Renzi; Acerbo, Flavia Sofia; Son, Tong Duy; Patrinos, Panagiotis
Imitation Learning from Observations: An Autoregressive Mixture of Experts Approach Working paper
2024.
@workingpaper{wang2024imitation,
title = {Imitation Learning from Observations: An Autoregressive Mixture of Experts Approach},
author = {Renzi Wang and Flavia Sofia Acerbo and Tong Duy Son and Panagiotis Patrinos},
url = {https://doi.org/10.48550/arXiv.2411.08232
},
year = {2024},
date = {2024-11-12},
abstract = {This paper presents a novel approach to imitation learning from observations, where an autoregressive mixture of experts model is deployed to fit the underlying policy. The parameters of the model are learned via a two-stage framework. By leveraging the existing dynamics knowledge, the first stage of the framework estimates the control input sequences and hence reduces the problem complexity. At the second stage, the policy is learned by solving a regularized maximum-likelihood estimation problem using the estimated control input sequences. We further extend the learning procedure by incorporating a Lyapunov stability constraint to ensure asymptotic stability of the identified model, for accurate multi-step predictions. The effectiveness of the proposed framework is validated using two autonomous driving datasets collected from human demonstrations, demonstrating its practical applicability in modelling complex nonlinear dynamics.},
keywords = {},
pubstate = {published},
tppubtype = {workingpaper}
}
Wang, Renzi; Bodard, Alexander; Schuurmans, Mathijs; Patrinos, Panagiotis
EM++: A parameter learning framework for stochastic switching systems Working paper
2024.
@workingpaper{wang2024em++,,
title = {EM++: A parameter learning framework for stochastic switching systems},
author = {Renzi Wang and Alexander Bodard and Mathijs Schuurmans and Panagiotis Patrinos},
url = {https://doi.org/10.48550/arXiv.2407.16359
},
year = {2024},
date = {2024-07-01},
abstract = {This paper proposes a general switching dynamical system model, and a custom majorization-minimization-based algorithm EM++ for identifying its parameters. For certain families of distributions, such as Gaussian distributions, this algorithm reduces to the well-known expectation-maximization method. We prove global convergence of the algorithm under suitable assumptions, thus addressing an important open issue in the switching system identification literature. The effectiveness of both the proposed model and algorithm is validated through extensive numerical experiments.},
keywords = {},
pubstate = {published},
tppubtype = {workingpaper}
}
Wang, Renzi; Schuurmans, Mathijs; Patrinos, Panagiotis
Interaction-aware Model Predictive Control for Autonomous Driving Proceedings Article
In: 2023 European Control Conference (ECC), pp. 1-6, IEEE, Bucharest, Romania, 2023, ISBN: 978-3-907144-08-4.
@inproceedings{Wang2023,
title = {Interaction-aware Model Predictive Control for Autonomous Driving},
author = {Renzi Wang and Mathijs Schuurmans and Panagiotis Patrinos},
url = {https://ieeexplore.ieee.org/document/10178332
https://arxiv.org/abs/2211.17053},
doi = {https://doi.org/10.23919/ECC57647.2023.10178332},
isbn = {978-3-907144-08-4},
year = {2023},
date = {2023-07-17},
urldate = {2023-07-17},
booktitle = {2023 European Control Conference (ECC)},
pages = {1-6},
publisher = {IEEE},
address = {Bucharest, Romania},
abstract = {We propose an interaction-aware stochastic model predictive control (MPC) strategy for lane merging tasks in automated driving. The MPC strategy is integrated with an online learning framework, which models a given driver’s cooperation level as an unknown parameter in a state-dependent probability distribution. The online learning framework adaptively estimates the surrounding vehicle’s cooperation level with the vehicle’s past state trajectory and combines this with a kinematic vehicle model to predict the distribution of a multimodal future state trajectory. Learning is conducted using logistic regression, enabling fast online computations. The multi-future prediction is used in the MPC algorithm to compute the optimal control input while satisfying safety constraints. We demonstrate our algorithm in an interactive lane changing scenario with drivers in different randomly selected cooperation levels.},
keywords = {},
pubstate = {published},
tppubtype = {inproceedings}
}