Flavia Acerbo
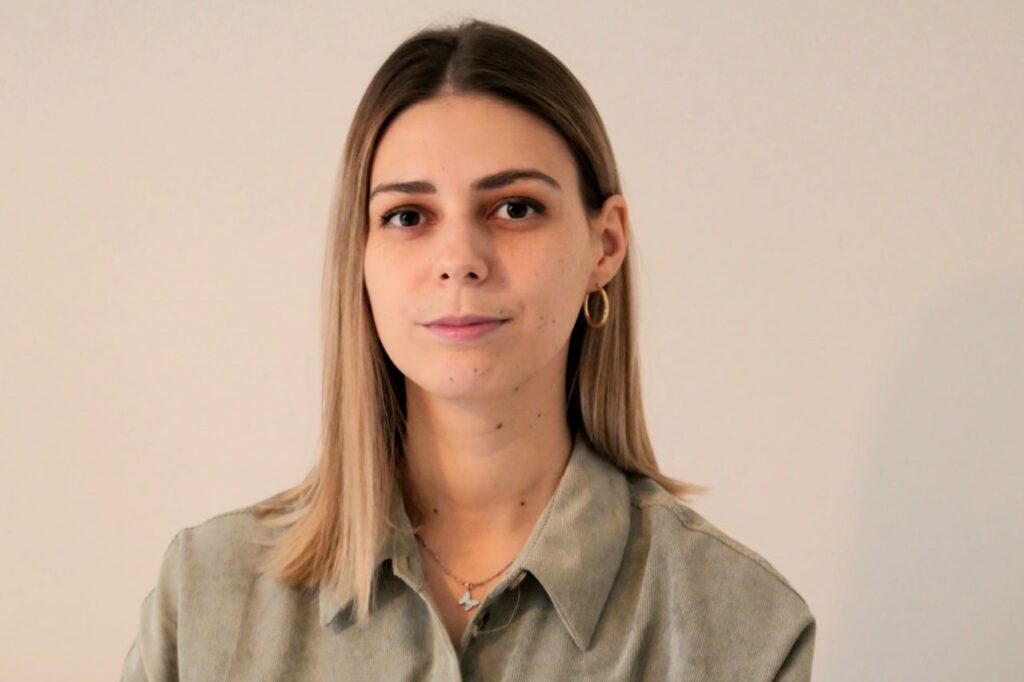
Flavia Sofia Acerbo is a researcher at Siemens Digital Industries Software, based in Leuven. She obtained her Bachelor’s degree in Computer Engineering in 2017 and her Master’s degree in Mechatronic Engineering in 2019, both cum laude and from Politecnico di Torino. After graduating, she joined Siemens Digital Industries Software, where she is currently working on a PhD project with KU Leuven on human imitation for autonomous driving comfort, which tries to develop methodologies to learn human-like controllers from driving data to improve passenger perception in automated vehicles. Her research interests lie in the intersection between optimal control and machine learning, specifically around learning-based Model Predictive Control and Reinforcement Learning.
Project description
The PhD project aims at developing a methodology to systematically include a human-like driving style into Advanced Driver Assistance Systems (ADAS) development, providing both safe and personalized driving. The study relies on demonstrations performed by the considered user and mixed-real virtual design and testing. The approach should be easily adapted to various automated driving tasks with minimal use of domain knowledge, automatically inferring the key parameters that describe the demonstrated driving style from high-dimensional multi-source datasets.
Publications
Wang, Renzi; Acerbo, Flavia Sofia; Son, Tong Duy; Patrinos, Panagiotis
Imitation Learning from Observations: An Autoregressive Mixture of Experts Approach Working paper
2024.
@workingpaper{wang2024imitation,
title = {Imitation Learning from Observations: An Autoregressive Mixture of Experts Approach},
author = {Renzi Wang and Flavia Sofia Acerbo and Tong Duy Son and Panagiotis Patrinos},
url = {https://doi.org/10.48550/arXiv.2411.08232
},
year = {2024},
date = {2024-11-12},
abstract = {This paper presents a novel approach to imitation learning from observations, where an autoregressive mixture of experts model is deployed to fit the underlying policy. The parameters of the model are learned via a two-stage framework. By leveraging the existing dynamics knowledge, the first stage of the framework estimates the control input sequences and hence reduces the problem complexity. At the second stage, the policy is learned by solving a regularized maximum-likelihood estimation problem using the estimated control input sequences. We further extend the learning procedure by incorporating a Lyapunov stability constraint to ensure asymptotic stability of the identified model, for accurate multi-step predictions. The effectiveness of the proposed framework is validated using two autonomous driving datasets collected from human demonstrations, demonstrating its practical applicability in modelling complex nonlinear dynamics.},
keywords = {},
pubstate = {published},
tppubtype = {workingpaper}
}
Acerbo, Flavia Sofia; Swevers, Jan; Tuytelaars, Tinne; Son, Tong Duy
Driving from Vision through Differentiable Optimal Control Proceedings Article
In: 2024 IEEE/RSJ International Conference on Intelligent Robots and Systems (IROS), pp. 2153-0866, IEEE, Abu Dhabi, United Arab Emirates, 2024, ISBN: 979-8-3503-7770-5.
@inproceedings{acerbo24drividoc,
title = {Driving from Vision through Differentiable Optimal Control},
author = {Flavia Sofia Acerbo and Jan Swevers and Tinne Tuytelaars and Tong Duy Son},
url = {https://doi.org/10.48550/arXiv.2403.15102
},
doi = {10.1109/IROS58592.2024.10802306},
isbn = {979-8-3503-7770-5},
year = {2024},
date = {2024-10-01},
booktitle = {2024 IEEE/RSJ International Conference on Intelligent Robots and Systems (IROS)},
pages = {2153-0866},
publisher = {IEEE},
address = {Abu Dhabi, United Arab Emirates},
abstract = {This paper proposes DriViDOC: a framework for Driving from Vision through Differentiable Optimal Control, and its application to learn autonomous driving controllers from human demonstrations. DriViDOC combines the automatic inference of relevant features from camera frames with the properties of nonlinear model predictive control (NMPC), such as constraint satisfaction. Our approach leverages the differentiability of parametric NMPC, allowing for end-to-end learning of the driving model from images to control. The model is trained on an offline dataset comprising various human demonstrations collected on a motion-base driving simulator. During online testing, the model demonstrates successful imitation of different driving styles, and the interpreted NMPC parameters provide insights into the achievement of specific driving behaviors. Our experimental results show that DriViDOC outperforms other methods involving NMPC and neural networks, exhibiting an average improvement of 20% in imitation scores.},
keywords = {},
pubstate = {published},
tppubtype = {inproceedings}
}
Acerbo, Flavia Sofia; Swevers, Jan; Tuytelaars, Tinne; Son, Tong Duy
Evaluation of MPC-based Imitation Learning for Human-like Autonomous Driving Proceedings Article
In: 22nd IFAC World Congress, pp. 4871-4876, Elsevier Ltd, Yokohama, Japan, 2023, ISSN: 2405-8963.
@inproceedings{acerboEvaluationMPCbasedImitation2023,
title = {Evaluation of MPC-based Imitation Learning for Human-like Autonomous Driving},
author = {Flavia Sofia Acerbo and Jan Swevers and Tinne Tuytelaars and Tong Duy Son},
url = {https://www.sciencedirect.com/science/article/pii/S2405896323016610},
doi = {https://doi.org/10.1016/j.ifacol.2023.10.1257},
issn = {2405-8963},
year = {2023},
date = {2023-11-30},
urldate = {2023-11-30},
booktitle = {22nd IFAC World Congress},
journal = { IFAC-PapersOnLine},
volume = {56},
number = {2},
pages = {4871-4876},
publisher = {Elsevier Ltd},
address = {Yokohama, Japan},
abstract = {This work evaluates and analyzes the combination of imitation learning (IL) and differentiable model predictive control (MPC) for the application of human-like autonomous driving. We combine MPC with a hierarchical learning-based policy, and measure its performance in open-loop and closed-loop with metrics related to safety, comfort and similarity to human driving characteristics. We also demonstrate the value of augmenting open-loop behavioral cloning with closed-loop training for a more robust learning, approximating the policy gradient through time with the state space model used by the MPC. We perform experimental evaluations on a lane keeping control system, learned from demonstrations collected on a fixed-base driving simulator, and show that our imitative policies approach the human driving style preferences.},
keywords = {},
pubstate = {published},
tppubtype = {inproceedings}
}
Acerbo, Flavia Sofia; Swevers, Jan; Tuytelaars, Tinne; Son, Tong Duy
MPC-based Imitation Learning for Safe and Human-like Autonomous Driving Workshop
2022.
@workshop{acerbo2022mpc,
title = {MPC-based Imitation Learning for Safe and Human-like Autonomous Driving},
author = {Flavia Sofia Acerbo and Jan Swevers and Tinne Tuytelaars and Tong Duy Son},
doi = {https://doi.org/10.48550/arXiv.2206.12348},
year = {2022},
date = {2022-01-01},
urldate = {2022-01-01},
journal = {arXiv preprint arXiv:2206.12348},
abstract = {To ensure user acceptance of autonomous vehicles (AVs), control systems are being developed to mimic human drivers from demonstrations of desired driving behaviors. Imitation learning (IL) algorithms serve this purpose, but struggle to provide safety guarantees on the resulting closed-loop system trajectories. On the other hand, Model Predictive Control (MPC) can handle nonlinear systems with safety constraints, but realizing human-like driving with it requires extensive domain knowledge. This work suggests the use of a seamless combination of the two techniques to learn safe AV controllers from demonstrations of desired driving behaviors, by using MPC as a differentiable control layer within a hierarchical IL policy. With this strategy, IL is performed in closed-loop and end-to-end, through parameters in the MPC cost, model or constraints. Experimental results of this methodology are analyzed for the design of a lane keeping control system, learned via behavioral cloning from observations (BCO), given human demonstrations on a fixed-base driving simulator.},
keywords = {},
pubstate = {published},
tppubtype = {workshop}
}