PhD Candidate in Information Technology – Systems and Control at Politecnico di Milano
ODYS Srl
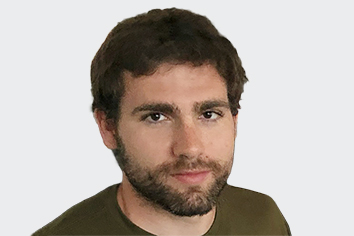
Kristoffer Løwenstein graduated from Aarhus University with a degree in mechanical engineering in January 2021. He worked with soft robotics in his thesis. His major research interests lie within dynamics, robotics, controls, artificial Intelligence, and the interplay between these fields. Kristoffer has carried out exchange semesters at Royal Melbourne Institute of Technology and Politecnico di Milano. Besides his studies, he played basketball at Bakken Bears winning multiple national championships in Denmark.
Project description
Next-generation embedded control systems will have to cope with tight constraints on computation and storage resources, while at the same time satisfying increasingly challenging requirements on performance quality, energy consumption, safety, emissions, etc. In this context, model-based control techniques such as MPC are envisioned to play a key role, by providing a systematic framework to minimize a desired objective function under constraints. Real application of online MPC to high-volume automotive production programs and other industrial applications has only very recently started to blossom and will likely continue to spread in the next years, but developments in the field of learning-based MPC, i.e. novel techniques nonlinear MPC incorporating machine learning techniques, is expected to accelerate the use even further.
This research project focuses on the development of novel physics-informed methods for learning and adapting prediction models for embedded nonlinear MPC. By exploiting physical knowledge in the training process learning-based components such as neural networks of rather limited size can be used to capture model mismatches. The developed algorithms aims at easing the design of MPC controllers and improve their performance, by reducing calibration time before production and adapting calibration parameters at run-time. Further, tailored optimization algorithms for solving the underlying numerical programs are investigated to enable real-time embedded applications of the proposed learning-based MPC methods.
Publications
Løwenstein, Kristoffer Fink; Bernardini, Daniele; Patrinos, Panagiotis
QPALM-OCP: A Newton-type Proximal Augmented Lagrangian tailored for Quadratic Programs arising in Model Predictive Control Journal Article
In: IEEE Control Systems Letters, 2024, ISSN: 2475-1456, (Submitted to the 63rd IEEE Conference on Decision and Control 2024 (CDC)).
@article{Lowenstein2024QPALMOCP,
title = {QPALM-OCP: A Newton-type Proximal Augmented Lagrangian tailored for Quadratic Programs arising in Model Predictive Control},
author = {Kristoffer Fink Løwenstein and Daniele Bernardini and Panagiotis Patrinos},
doi = {10.1109/LCSYS.2024.3410638},
issn = {2475-1456},
year = {2024},
date = {2024-06-06},
urldate = {2024-04-02},
journal = {IEEE Control Systems Letters},
abstract = {In Model Predictive Control (MPC) fast and reliable Quadratic Programming (QP) solvers are of fundamental importance. The inherent structure of the subsequent Optimal Control Problems (OCPs) can lead to substantial performance improvements if exploited. Therefore, we present a structure-exploiting proximal augmented Lagrangian based solver extending the general-purpose QP-solver QPALM. Our solver relies on semismooth Newton iterates to solve the inner sub-problem while directly accounting for the OCP structure via efficient and sparse matrix factorizations. The matrices to be factorized depends on the active set and therefore low-rank factorization updates can be employed like in active-set methods resulting in cheap iterates. We benchmark our solver against other state-of-the-art QP-solvers and our algorithm compare favorably against these solvers},
note = {Submitted to the 63rd IEEE Conference on Decision and Control 2024 (CDC)},
keywords = {},
pubstate = {published},
tppubtype = {article}
}
Løwenstein, Kristoffer Fink; Fagiano, Lorenzo; Bernardini, Daniele; Bemporad, Alberto
Physics-Informed Online Learning by Moving Horizon Estimation: Learning Recurrent Neural Networks in Gray-box Models Proceedings Forthcoming
Forthcoming, (Accepted to the 2024 IFAC Conference on Nonlinear Model Predictive Control (NMPC)).
@proceedings{Lowenstein2024PhysicsInformedRNN,
title = {Physics-Informed Online Learning by Moving Horizon Estimation: Learning Recurrent Neural Networks in Gray-box Models},
author = {Kristoffer Fink Løwenstein and Lorenzo Fagiano and Daniele Bernardini and Alberto Bemporad},
year = {2024},
date = {2024-04-02},
abstract = {In Model Predictive Control (MPC) closed-loop performance heavily depends on the quality of the underlying prediction model, where such a model must be accurate and yet
simple. A key feature in modern MPC applications is the potential for online model adaptation to cope with time-varying changes, part-to-part variations, and complex features of the system dynamics not caught by models derived from first principles. In this paper, we propose to use a physics-informed, or gray-box, model that extends the physics-based model with a data-driven component, namely a Recurrent Neural Network (RNN). Relying on physics-informed models allows for a rather limited size of the RNN, thereby enhancing online applicability compared to pure black-box models. This work presents a method based on Moving Horizon Estimation (MHE) for simultaneous state estimation and learning of the RNN sub-model, a potentially challenging issue due to limited information available in noisy input output data and lack of knowledge of the internal state of the RNN. We provide a case study on a quadruple tank benchmark showing how the method can cope with part-to-part variations.},
note = {Accepted to the 2024 IFAC Conference on Nonlinear Model Predictive Control (NMPC)},
keywords = {},
pubstate = {forthcoming},
tppubtype = {proceedings}
}
simple. A key feature in modern MPC applications is the potential for online model adaptation to cope with time-varying changes, part-to-part variations, and complex features of the system dynamics not caught by models derived from first principles. In this paper, we propose to use a physics-informed, or gray-box, model that extends the physics-based model with a data-driven component, namely a Recurrent Neural Network (RNN). Relying on physics-informed models allows for a rather limited size of the RNN, thereby enhancing online applicability compared to pure black-box models. This work presents a method based on Moving Horizon Estimation (MHE) for simultaneous state estimation and learning of the RNN sub-model, a potentially challenging issue due to limited information available in noisy input output data and lack of knowledge of the internal state of the RNN. We provide a case study on a quadruple tank benchmark showing how the method can cope with part-to-part variations.
Meza, Gonzalo; Løwenstein, Kristoffer Fink; Fagiano, Lorenzo
Obstacle avoidance for a robotic manipulator with linear-quadratic Model Predictive Control Proceedings Article Forthcoming
In: 2024 IEEE 20th International Conference on Automation Science and Engineering (CASE 2024), Forthcoming.
@inproceedings{Meza2024ObstacleMPC,
title = {Obstacle avoidance for a robotic manipulator with linear-quadratic Model Predictive Control},
author = {Gonzalo Meza and Kristoffer Fink Løwenstein and Lorenzo Fagiano },
year = {2024},
date = {2024-04-02},
urldate = {2024-04-02},
booktitle = {2024 IEEE 20th International Conference on Automation Science and Engineering (CASE 2024)},
abstract = {The problem of moving a six-degrees-of-freedom manipulator in an environment with unknown obstacles is considered. The manipulator is assumed to be equipped with an exteroceptive sensor that provides a partial sampling of the surroundings. A hierarchical control layout is proposed: in the outer layer, a path planner generates an obstacle free trajectory based on the available local information; in the inner layer, a Model-Predictive Controller formulated in the joint space tracks the trajectory while reactively avoiding unseen obstacles at a higher rate. By constructing a polytopic under-approximation of the free environment end employing a suitable estimate of the Jacobian matrix of the manipulator, the predictive controller features a convex quadratic cost and linear constraints, thus requiring the solution of a quadratic program at each time step. The proposed method is evaluated on the kinematic model of a MyCobot280 robotic arm, showing the potential for real-time feasibility.},
keywords = {},
pubstate = {forthcoming},
tppubtype = {inproceedings}
}
Løwenstein, Kristoffer Fink; Fagiano, Lorenzo; Bernardini, Daniele; Bemporad, Alberto
Physics-Informed Online Learning of Gray-box Models by Moving Horizon Estimation Journal Article
In: European Journal of Control, pp. 100861, 2023, ISSN: 0947-3580.
@article{Lowenstein2023PhysicsInformed,
title = {Physics-Informed Online Learning of Gray-box Models by Moving Horizon Estimation},
author = {Kristoffer Fink Løwenstein and Lorenzo Fagiano and Daniele Bernardini and Alberto Bemporad},
url = {https://www.sciencedirect.com/science/article/pii/S0947358023000900},
doi = {10.1016/j.ejcon.2023.100861},
issn = {0947-3580},
year = {2023},
date = {2023-07-03},
urldate = {2023-07-03},
journal = {European Journal of Control},
pages = {100861},
abstract = {A simple yet expressive prediction model is an essential ingredient in model-based control and estimation. Models derived from fundamental physical principles may fail to capture the complexity of the actual system dynamics. A potential solution is the use of a physics-informed, or gray-box model that extends a physics-based model with a data-driven part. Learning the latter might be challenging, due to noisy measurements and lack of full state information. This work presents a method based on Moving Horizon Estimation (MHE) for simultaneous state estimation and training of a black-box submodel, such as a neural network. The method can be used in offline training or applied online for adaptation without any prior knowledge than the white-box submodel. We analyze the capabilities of the method in a two degree of freedom robotic manipulator case study, also showing how it can be used for online adaptation to cope with a time-varying model mismatch.},
keywords = {},
pubstate = {published},
tppubtype = {article}
}