Research Engineer Associate and PhD candidate in Engineering Sciences at KU Leuven
Siemens Digital Industries Software
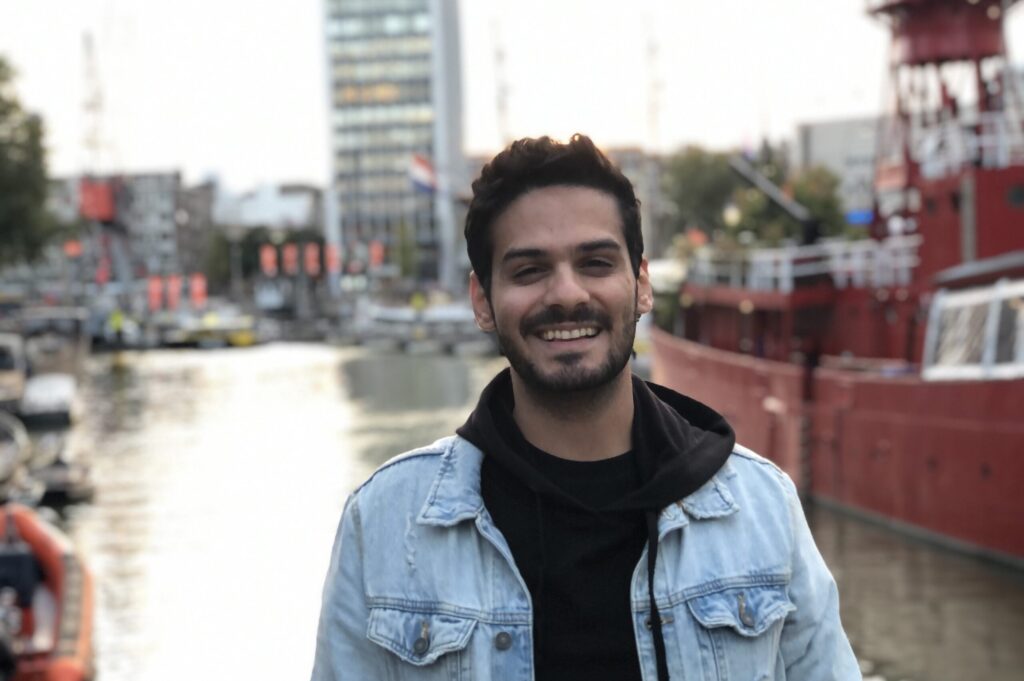
Jean Pierre Allamaa is a Lebanese student who graduated with a Masters in Mechanical Engineering with a specialization in mechatronics and control from Ecole Polytechnique Federale de Lausanne, Switzerland (EPFL) in 2020. His master thesis was about development and deployment of real-time optimal control for autonomous driving on road vehicles. Passionate about racing sports, he worked from September 2020 to June 2021 as a researcher in the Laboratoire d’Automatique (LA, EPFL) on real-time NMPC and embedded optimization for competitive autonomous racing.
Project description
Jean Pierre is working towards a novel methodogical and embedded implementation development, which combines both optimal control enhanced by learning and data to increase the Autonomous Driving (AD) acceptability and safety. The work considers advanced optimization and machine learning methods that are able to address challenging safety and comfort problems in autonomous driving. Safety is considered as the main driver for AD and ADAS development. While several existing assistance functionalities have proven their capabilities in simple use cases (e.g. adaptive cruise control), the automotive industry is continuously dealing with more safety-critical scenarios such as emergency lane change and intersection crossing. Today, most common control designs in the automotive industry rely on model-based non-optimal methods. They sometimes struggle to deal with safety-critical scenarios. Nonlinear model predictive control is one possible strategy that can deal with such safety concerns, however, it faces the challenge of real-time performance.
Recently, machine learning techniques have shown advantages in several AD control applications, in terms of comfort and scalability to complex scenarios. Importantly, they rely on data and can recover from degrading performances that model-based approaches suffer from. However, they show limitations due to their lack of fundamental and rigorous results on explainability, safety and stability. Therefore, this PhD will focus on combining the strengths of both optimal control and machine learning for embedded autonomous driving applications, to facilitate the development, deployment and adoption of autonomous driving control strategies.
Publications
Allamaa, Jean Pierre; Patrinos, Panagiotis; Ohtsuka, Toshiyuki; Son, Tong Duy
Real-time MPC with Control Barrier Functions for Autonomous Driving using Safety Enhanced Collocation Best Paper Proceedings Article
In: 8th IFAC Conference on Nonlinear Model Predictive Control NMPC 2024, pp. 392-399, IFAC-PapersOnLine, Kyoto, Japan, 2024, (Preprint: https://doi.org/10.48550/arXiv.2401.06648).
@inproceedings{allamaa2024RTMPCCBF,
title = {Real-time MPC with Control Barrier Functions for Autonomous Driving using Safety Enhanced Collocation},
author = {Jean Pierre Allamaa and Panagiotis Patrinos and Toshiyuki Ohtsuka and Tong Duy Son},
url = {https://www.sciencedirect.com/science/article/pii/S240589632401437X},
doi = {https://doi.org/10.1016/j.ifacol.2024.09.058},
year = {2024},
date = {2024-09-25},
urldate = {2024-09-25},
booktitle = {8th IFAC Conference on Nonlinear Model Predictive Control NMPC 2024},
volume = {58},
number = {18},
pages = {392-399},
publisher = {IFAC-PapersOnLine},
address = {Kyoto, Japan},
abstract = {The autonomous driving industry is continuously dealing with safety-critical scenarios, and nonlinear model predictive control (NMPC) is a powerful control strategy for handling such situations. However, standard safety constraints are not scalable and require a long NMPC horizon. Moreover, the adoption of NMPC in the automotive industry is limited by the heavy computation of numerical optimization routines. To address those issues, this paper presents a real-time capable NMPC for automated driving in urban environments, using control barrier functions (CBFs). Furthermore, the designed NMPC is based on a novel collocation transcription approach, named RESAFE/COL, that allows to reduce the number of optimization variables while still guaranteeing the continuous time (nonlinear) inequality constraints satisfaction, through regional convex hull approximation. RESAFE/COL is proven to be 5 times faster than multiple shooting and more tractable for embedded hardware without a decrease in the performance, nor accuracy and safety of the numerical solution. We validate our NMPC-CBF with RESAFE/COL on digital twins of the vehicle and the urban environment and show the safe controller's ability to improve crash avoidance by 91%. Supplementary visual material can be found at https://youtu.be/_EnbfYwljp4.},
note = {Preprint: https://doi.org/10.48550/arXiv.2401.06648},
keywords = {},
pubstate = {published},
tppubtype = {inproceedings}
}
Allamaa, Jean Pierre; Patrinos, Panagiotis; Auweraer, Herman Van; Son, Tong Duy
Learning-Based NMPC Adaptation for Autonomous Driving Using Parallelized Digital Twin Journal Article
In: IEEE Transactions on Control Systems Technology, vol. Early Access, pp. 1-16, 2024, ISSN: 1063-6536, (Preprint: https://doi.org/10.48550/arXiv.2402.16645).
@article{allamaa2024lbMPC,
title = {Learning-Based NMPC Adaptation for Autonomous Driving Using Parallelized Digital Twin},
author = {Jean Pierre Allamaa and Panagiotis Patrinos and Herman Van Auweraer and Tong Duy Son},
doi = {10.1109/TCST.2024.3437163},
issn = {1063-6536},
year = {2024},
date = {2024-08-14},
urldate = {2024-05-29},
journal = {IEEE Transactions on Control Systems Technology},
volume = {Early Access},
pages = {1-16},
abstract = {In this work, we focus on the challenge of transferring an autonomous driving (AD) controller from simulation to reality (Sim2Real). We propose a data-efficient method for online and on-the-fly adaptation of parametrizable control architectures such that the target closed-loop performance is optimized while accounting for uncertainties such as model mismatches, changes in the environment, and task variations. The novelty of the approach resides in leveraging black-box optimization enabled by executable digital twins (xDTs) for data-driven parameter calibration through derivative-free methods to directly adapt the controller in real time (RT). The xDTs are augmented with domain randomization (DR) for robustness and allow for safe parameter exploration. The proposed method requires a minimal amount of interaction with the real world as it pushes the exploration toward the xDTs. We validate our approach through real-world experiments, demonstrating its effectiveness in transferring and fine-tuning a nonlinear model predictive control (NMPC) with nine parameters, in under 10 min. This eliminates the need for hours-long manual tuning and lengthy machine learning training and data collection phases. Our results show that the online adapted NMPC directly compensates for the Sim2Real gap and avoids overtuning in simulation. Importantly, a 75% improvement in tracking performance is achieved, and the Sim2Real gap over the target performance is reduced from a factor of 876 to 1.033.},
note = {Preprint: https://doi.org/10.48550/arXiv.2402.16645},
keywords = {},
pubstate = {published},
tppubtype = {article}
}
Voogd, Kevin; Allamaa, Jean Pierre; Alonso-Mora, Javier; Son, Tong Duy
Reinforcement Learning from Simulation to Real World Autonomous Driving using Digital Twin Proceedings Article
In: 22nd IFAC World Congress 2023, pp. 1510-1515, Elsevier Ltd, Yokohama, Japan, 2023, ISSN: 2405-8963.
@inproceedings{Voogd2023ReinforcementLF,
title = {Reinforcement Learning from Simulation to Real World Autonomous Driving using Digital Twin},
author = {Kevin Voogd and Jean Pierre Allamaa and Javier Alonso-Mora and Tong Duy Son},
url = {https://www.sciencedirect.com/science/article/pii/S2405896323022553},
doi = {https://doi.org/10.1016/j.ifacol.2023.10.1846},
issn = {2405-8963},
year = {2023},
date = {2023-11-30},
urldate = {2023-11-30},
booktitle = {22nd IFAC World Congress 2023},
journal = {IFAC-PapersOnLine},
volume = {56},
number = {2},
pages = {1510-1515},
publisher = {Elsevier Ltd},
address = {Yokohama, Japan},
abstract = {Reinforcement learning (RL) is a promising solution for autonomous vehicles to deal with complex and uncertain traffic environments. The RL training process is however expensive, unsafe, and time-consuming. Algorithms are often developed first in simulation and then transferred to the real-world, leading to a common sim2real challenge where performance decreases when the domain changes. In this paper, we propose a transfer learning process to minimize the gap by exploiting digital twin technology, relying on a systematic and simultaneous combination of virtual and real world data coming from vehicle dynamics and traffic scenarios. The model and testing environment is evolved from model, hardware to vehicle in the loop and proving ground testing stages, similar to standard development cycle in the automotive industry. In particular, we also integrate other transfer learning techniques such as domain randomization and adaptation in each stage. The simulation and real data are gradually incorporated to accelerate and make the transfer learning process more robust. The proposed RL methodology is applied to develop a path-following steering controller for an autonomous electric vehicle. After learning and deploying the real-time RL control policy on the vehicle, we obtained satisfactory and safe control performance already from the first deployment, demonstrating the advantages of the proposed digital twin based learning process.
},
keywords = {},
pubstate = {published},
tppubtype = {inproceedings}
}
Allamaa, Jean Pierre; Patrinos, Panagiotis; Auweraer, Herman Van; Son, Tong Duy
Safety Envelope for Orthogonal Collocation Methods in Embedded Optimal Control Proceedings Article
In: 2023 European Control Conference (ECC), pp. 1-7, IEEE, Bucharest, Romania, 2023, ISBN: 978-3-907144-08-4.
@inproceedings{Allamaa2022SafetyEF,
title = {Safety Envelope for Orthogonal Collocation Methods in Embedded Optimal Control},
author = {Jean Pierre Allamaa and Panagiotis Patrinos and Herman Van Auweraer and Tong Duy Son},
url = {https://ieeexplore.ieee.org/document/10178116
https://arxiv.org/abs/2211.14853},
doi = {https://doi.org/10.23919/ECC57647.2023.10178116},
isbn = {978-3-907144-08-4},
year = {2023},
date = {2023-07-17},
urldate = {2023-07-17},
booktitle = {2023 European Control Conference (ECC)},
pages = {1-7},
publisher = {IEEE},
address = {Bucharest, Romania},
abstract = {Orthogonal collocation methods are direct approaches for solving optimal control problems (OCP). A high solution accuracy is achieved with few optimization variables, making it more favorable for embedded and real-time NMPC applications. However, collocation approaches lack a guarantee about the safety of the resulting trajectory as inequality constraints are only set on a finite number of collocation points. In this paper we propose a method to efficiently create a convex safety envelope containing the trajectory such that the solution fully satisfies the OCP constraints. We make use of Bernstein approximations of a polynomial’s extrema and span the solution over an orthogonal basis using Legendre polynomials. The tightness of the safety envelope estimation, high accuracy in solving the underlying differential equations, fast rate of convergence and little conservatism are properties of the presented approach making it a suitable method for safe real-time NMPC deployment. We show that our method has comparable computational performance to pseudospectral approaches and can accurately approximate the original OCP up to 9 times more quickly than standard multiple-shooting method in autonomous driving applications, without adding complexity to the formulation.},
keywords = {},
pubstate = {published},
tppubtype = {inproceedings}
}
Allamaa, Jean Pierre; Listov, Petr; Auweraer, Herman Van; Jones, Colin; Son, Tong Duy
Real-time Nonlinear MPC Strategy with Full Vehicle Validation for Autonomous Driving Proceedings Article
In: 2022 American Control Conference (ACC), pp. 1982-1987, IEEE, Atlanta, GA, USA, 2022, ISBN: 978-1-6654-5196-3.
@inproceedings{Allamaa2022RTNMPCStrategy,
title = {Real-time Nonlinear MPC Strategy with Full Vehicle Validation for Autonomous Driving},
author = {Jean Pierre Allamaa and Petr Listov and Herman Van Auweraer and Colin Jones and Tong Duy Son},
url = {https://ieeexplore.ieee.org/document/9867514},
doi = {10.23919/ACC53348.2022.9867514},
isbn = {978-1-6654-5196-3},
year = {2022},
date = {2022-06-09},
booktitle = {2022 American Control Conference (ACC)},
pages = {1982-1987},
publisher = {IEEE},
address = {Atlanta, GA, USA},
abstract = {In this paper, we present the development and deployment of an embedded optimal control strategy for autonomous driving applications on a Ford Focus road vehicle. Non-linear model predictive control (NMPC) is designed and deployed on a system with hard real-time constraints. We show the properties of sequential quadratic programming (SQP) optimization solvers that are suitable for driving tasks. Importantly, the designed algorithms are validated based on a standard automotive XiL development cycle: model-in-the-loop (MiL) with high fidelity vehicle dynamics, hardware-in-the-loop (HiL) with vehicle actuation and embedded platform, and full vehicle-hardware-in-the-loop (VeHiL). The autonomous driving environment contains both virtual simulation and physical proving ground tracks. NMPC algorithms and optimal control problem formulation are fine-tuned using a deployable C code via code generation compatible with the target embedded toolchains. Finally, the developed systems are applied to autonomous collision avoidance, trajectory tracking, and lane change at high speed on city/highway and low speed at a parking environment.},
keywords = {},
pubstate = {published},
tppubtype = {inproceedings}
}
Allamaa, Jean Pierre; Patrinos, Panagiotis; Auweraer, Herman Van; Son, Tong Duy
Sim2real for Autonomous Vehicle Control using Executable Digital Twin Proceedings Article
In: 10th IFAC Symposium on Advances in Automotive Control (AAC), pp. 385-391, Elsevier Ltd, 2022, ISSN: 2405-8963.
@inproceedings{ALLAMAA2022385,
title = {Sim2real for Autonomous Vehicle Control using Executable Digital Twin},
author = {Jean Pierre Allamaa and Panagiotis Patrinos and Herman Van Auweraer and Tong Duy Son},
url = {https://www.sciencedirect.com/science/article/pii/S2405896322023461},
doi = {https://doi.org/10.1016/j.ifacol.2022.10.314},
issn = {2405-8963},
year = {2022},
date = {2022-01-01},
urldate = {2022-01-01},
booktitle = {10th IFAC Symposium on Advances in Automotive Control (AAC)},
journal = {IFAC-PapersOnLine},
volume = {55},
number = {24},
pages = {385-391},
publisher = {Elsevier Ltd},
abstract = {In this work, we propose a sim2real method to transfer and adapt a nonlinear model predictive controller (NMPC) from simulation to the real target system based on executable digital twin (xDT). The xDT model is a high fidelity vehicle dynamics simulator, executable online in the control parameter randomization and learning process. The parameters are adapted to gradually improve control performance and deal with changing real-world environment. In particular, the performance metric is not required to be differentiable nor analytical with respect to the control parameters and system dynamics are not necessary linearized. Eventually, the proposed sim2real framework leverages altogether online high fidelity simulator, data-driven estimations, and simulation based optimization to transfer and adapt efficiently a controller developed in simulation environment to the real platform. Our experiment demonstrates that a high control performance is achieved without tedious time and labor consuming tuning.},
keywords = {},
pubstate = {published},
tppubtype = {inproceedings}
}