PhD candidate in Robotics, Control and Intelligent Systems
PREDICT group, Automatic Control Laboratory
EPFL
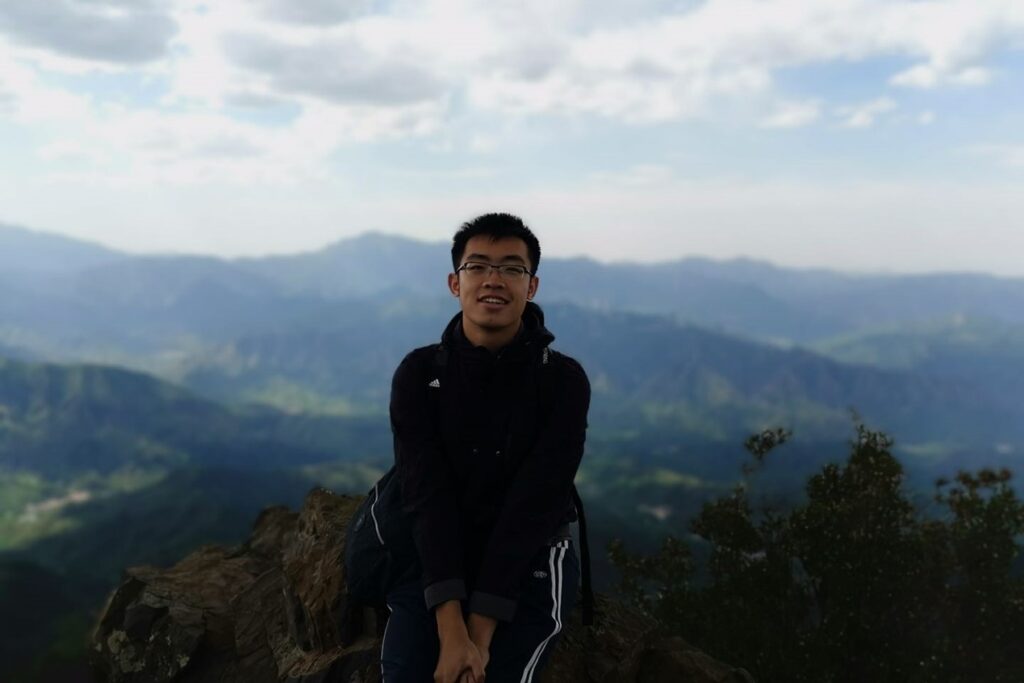
Shaohui Yang was born in Beijing, China in 1997. He obtained his B.Eng. degree in computer science from the Hong Kong University of Science and Technology (HKUST), China in 2019, and his M.S. degree in Systems, Control, and Robotics from KTH Royal Institute of Technology, Sweden in 2021. Conducted in the Robotics Systems Lab (RSL) at ETH Zurich, his master thesis focused on nonlinear model predictive control, trajectory optimization, and generation for quadrupedal robots. He has experience in a pipeline of mobile robotics research aspects including estimation, perception, planning, and control, and various robotics hardware platforms including quadrotors, legged robots, and autonomous vehicles.
Project description
While the performance and potential of nonlinear model predictive control have been widely demonstrated, two main challenges still dominate 1. theoretical guarantee of NMPC; 2. online computational burden. The latter is the key limiting factor for realizing fast NMPC in real-world applications.
This PhD project aims to tackle the above issues by exploiting structures of nonlinear predictive control, from both modeling and optimization perspectives. Specialties of models will enhance the theoretical properties of NMPC and provide sub-optimal solutions at much lower costs under reasonable manipulation. Stagewise structure of the optimal control problems leads to efficient solvers from the optimization aspect.
Possible research directions include the development of tailored real-time optimization routines exploiting model structures and parallelism on GPU at the same time, lossless convexification, or approximate convex formulation of nonlinear optimal control problems with certificates, as well as application of the above to robotics platforms.
Publications
Sorry, no publications matched your criteria.