PhD Candidate
Knowledge Engineering & Digital Twin Technologies
Robert Bosch GmbH, Corporate Research
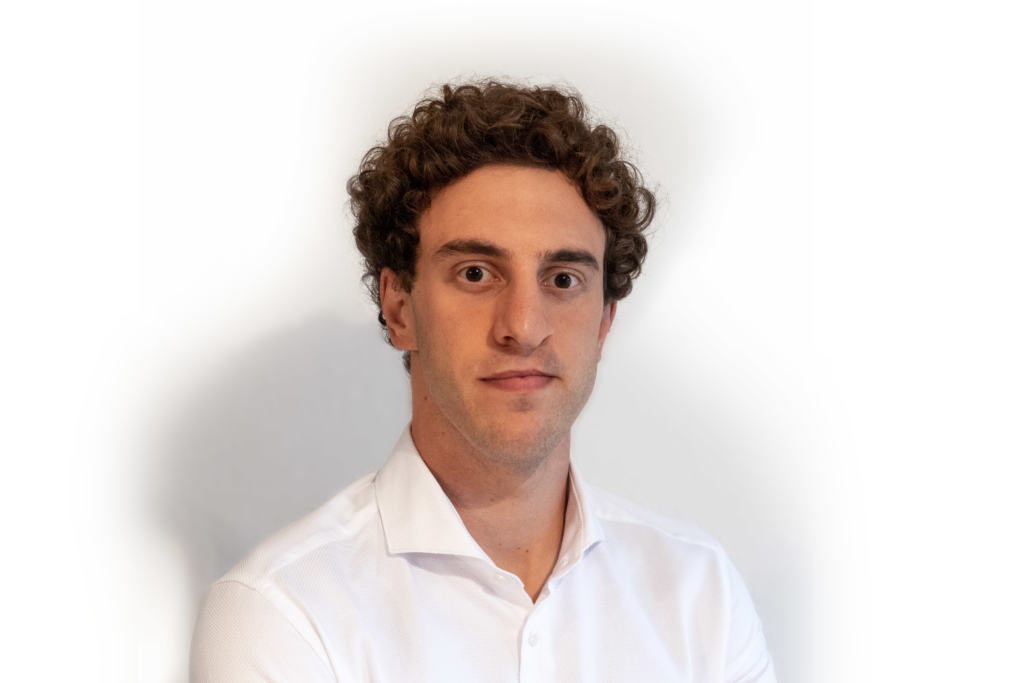
Leonardo Cecchin graduated in Automation and Control Engineering at Politecnico di Milano in October 2020, with the thesis “Graph-Based Exploration and Mapping for Mobile Robots”. From November 2020 until June 2021 he has been a Research Fellow at SAS-Lab, working on graph-based approaches for exploration and mapping with autonomous multicopter drones, equipped with positioning systems and LiDAR turrets. Since July 2021 he is carrying out a PhD at Bosch Research, Germany, in the framework of the Marie Curie Initial Training Network “ELO-X”.
Hydraulic systems are integral to our modern society, powering a wide range of applications, from industrial machinery to construction equipment. Their efficiency is vital in conserving energy resources. To address this, the project centers on the creation of an Adaptive Multilayer Model Predictive Controller for Hydraulic systems. It entails modeling of the system, the exploration of diverse control strategies across system components, and the holistic assessment of the controller’s architecture. The controller’s performance is rigorously tested through simulation, benchmarked against alternative approaches, and ultimately validated in real-world experimental settings.
Publications
Cecchin, Leonardo; Ohtsuka, Toshiyuki; Trachte, Adrian; Diehl, Moritz
Model Predictive Controller for Hydraulic Cylinders with Independent Metering Control Valves Working paper
2024, (Submitted to the 2024 IFAC Conference on Nonlinear Model Predictive Control (NMPC)).
@workingpaper{cecchin2024imc,
title = {Model Predictive Controller for Hydraulic Cylinders with Independent Metering Control Valves},
author = {Leonardo Cecchin and Toshiyuki Ohtsuka and Adrian Trachte and Moritz Diehl},
year = {2024},
date = {2024-02-12},
urldate = {2024-02-12},
abstract = {Hydraulic cylinders are pivotal components in various industrial, construction, and off-highway applications, where efficient actuation is crucial for reducing energy consumption, minimizing heat generation, and extending components' lifespan. The integration of Independent Metering Control, a valve topology allowing five valves to independently control the flow, represents a significant advancement in enhancing hydraulic systems' performance. However, the lack of a reliable and flexible control solution remains a challenge. In this paper, we present the implementation of nonlinear Model Predictive Control, using a favorable model formulation and a state-of-the-art solver. We show how it can deliver close-to-optimal performance with real-time capabilities, addressing the current gap in achieving efficient control for hydraulic cylinders with Independent Metering Control.},
note = {Submitted to the 2024 IFAC Conference on Nonlinear Model Predictive Control (NMPC)},
keywords = {},
pubstate = {published},
tppubtype = {workingpaper}
}
Cecchin, Leonardo; Trachte, Adrian; Fagiano, Lorenzo; Diehl, Moritz
Real-time prediction of human-generated reference signals: a case study in advanced digging control Working paper
2024, (Submitted to the 2024 European Control Conference (ECC)).
@workingpaper{cecchin2024pred,
title = {Real-time prediction of human-generated reference signals: a case study in advanced digging control},
author = {Leonardo Cecchin and Adrian Trachte and Lorenzo Fagiano and Moritz Diehl},
year = {2024},
date = {2024-02-12},
urldate = {2024-02-12},
abstract = {Techniques like Model Predictive Control (MPC) and two-degrees-of-freedom structures (feedforward plus feedback) can improve the control performance by exploiting a prediction of the reference trajectory, which is assumed to be available. This assumption holds true when pre-defined reference trajectories are known a-priori, e.g. constant or piecewise linear, but fails in applications where a human operator chooses the reference at runtime. To cope with this problem, we study different approaches to use the collected data of human-generated reference signals to learn a predictive model of the operator commands. The considered methods are function approximation techniques based on Kriging, Set-Membership, and LSTM Neural Networks.
We summarize the principles and the implementation of each method, and compare their performance using an experimental data-set of operations from a real-world excavator, where four operator-defined reference signals are predicted.},
note = {Submitted to the 2024 European Control Conference (ECC)},
keywords = {},
pubstate = {published},
tppubtype = {workingpaper}
}
We summarize the principles and the implementation of each method, and compare their performance using an experimental data-set of operations from a real-world excavator, where four operator-defined reference signals are predicted.
Cecchin, Leonardo; Frey, Jonathan; Gering, Stefan; Manderla, Maximilian; Trachte, Adrian; Diehl, Moritz
Nonlinear Model Predictive Control for Efficient Control of Variable Speed Variable Displacement Pumps Proceedings Article
In: 2023 Modeling, Estimation and Control Conference (MECC), pp. 1–6, IFAC 2023.
@inproceedings{cecchin2023nonlinear,
title = {Nonlinear Model Predictive Control for Efficient Control of Variable Speed Variable Displacement Pumps},
author = { Leonardo Cecchin and Jonathan Frey and Stefan Gering and Maximilian Manderla and Adrian Trachte and Moritz Diehl},
year = {2023},
date = {2023-01-01},
urldate = {2023-01-01},
booktitle = {2023 Modeling, Estimation and Control Conference (MECC)},
pages = {1–6},
organization = {IFAC},
abstract = {Hydraulic pumps are a key component in manufacturing industry and off-highway vehicles.
Paired with diesel engines or electric motors, they provide hydraulic flow that can conveniently be used to power a variety of actuators.
Hydraulic power transmission has numerous advantages, unfortunately energy efficiency is usually not one of those.
The use of Variable Speed Variable Displacement pumps has been proven to be advantageous with respect to constant speed or constant displacement solutions: It allows to achieve higher efficiency and faster flow tracking dynamics.
This paper presents the development of a Model Predictive Control for this system, considering the nonlinearities and look-up-tables that characterize the system dynamics.
The Model Predictive Controller is then compared both in simulation and on test bench with a reference controller for such system, showing potential both regarding efficiency and flow tracking dynamics.},
keywords = {},
pubstate = {published},
tppubtype = {inproceedings}
}
Paired with diesel engines or electric motors, they provide hydraulic flow that can conveniently be used to power a variety of actuators.
Hydraulic power transmission has numerous advantages, unfortunately energy efficiency is usually not one of those.
The use of Variable Speed Variable Displacement pumps has been proven to be advantageous with respect to constant speed or constant displacement solutions: It allows to achieve higher efficiency and faster flow tracking dynamics.
This paper presents the development of a Model Predictive Control for this system, considering the nonlinearities and look-up-tables that characterize the system dynamics.
The Model Predictive Controller is then compared both in simulation and on test bench with a reference controller for such system, showing potential both regarding efficiency and flow tracking dynamics.
Cecchin, Leonardo; Baumgärtner, Katrin; Gering, Stefan; Diehl, Moritz
Locally Weighted Regression with Approximate Derivatives for Data-based optimization Proceedings Article
In: 2023 European Control Conference (ECC), pp. 1–6, IEEE 2023.
@inproceedings{cecchin2023locally,
title = {Locally Weighted Regression with Approximate Derivatives for Data-based optimization},
author = { Leonardo Cecchin and Katrin Baumgärtner and Stefan Gering and Moritz Diehl},
year = {2023},
date = {2023-01-01},
urldate = {2023-01-01},
booktitle = {2023 European Control Conference (ECC)},
pages = {1–6},
organization = {IEEE},
abstract = {Interpolation and approximation of data provided in terms of a Look-Up Table (LUT) is a common and well-known task, and is especially relevant for industrial applications. When using the function for point-wise evaluation, the method choice only affects the accuracy of the function value itself. However, when the LUT is used as part of an optimization problem formulation, a bad method choice can prevent convergence or alter significantly the outcome of the solver. Moreover, computational efficiency becomes critical due to the much higher number of evaluations required. This work focuses on a variation of Locally Weighted Regression, with approximate derivatives computation. The result is a method that allows one to obtain the function value together with the first n derivatives, at a reduced computational cost. Theoretical properties of the approach are analyzed, and the results of a minimization problem using the proposed method are compared with more traditional ones. The new approach shows promising performance and results, both for computational efficiency and effectiveness when used in optimization.},
keywords = {},
pubstate = {published},
tppubtype = {inproceedings}
}
Saccani, Danilo; Cecchin, Leonardo; Fagiano, Lorenzo
Multitrajectory Model Predictive Control for Safe UAV Navigation in an Unknown Environment Journal Article
In: IEEE Transactions on Control Systems Technology, pp. 1-16, 2022.
@article{9938397,
title = {Multitrajectory Model Predictive Control for Safe UAV Navigation in an Unknown Environment},
author = {Danilo Saccani and Leonardo Cecchin and Lorenzo Fagiano},
doi = {10.1109/TCST.2022.3216989},
year = {2022},
date = {2022-01-01},
urldate = {2022-01-01},
journal = {IEEE Transactions on Control Systems Technology},
pages = {1-16},
abstract = {The problem of navigating an unmanned aerial vehicle (UAV) in an unknown environment is addressed with a novel model predictive control (MPC) formulation, named multitrajectory MPC (mt-MPC). The objective is to safely drive the vehicle to the desired target location by relying only on the partial description of the surroundings provided by an exteroceptive sensor. This information results in time-varying constraints during the navigation among obstacles. The proposed mt-MPC generates a sequence of position set points that are fed to control loops at lower hierarchical levels. To do so, the mt-MPC predicts two different state trajectories, a safe one and an exploiting one, in the same finite horizon optimal control problem (FHOCP). This formulation, particularly suitable for problems with uncertain time-varying constraints, allows one to partially decouple constraint satisfaction (safety) from cost function minimization (exploitation). Uncertainty due to modeling errors and sensors noise is taken into account as well, in a set membership (SM) framework. Theoretical guarantees of persistent obstacle avoidance are derived under suitable assumptions, and the approach is demonstrated experimentally out-of-the-laboratory on a prototype built with off-the-shelf components.},
keywords = {},
pubstate = {published},
tppubtype = {article}
}