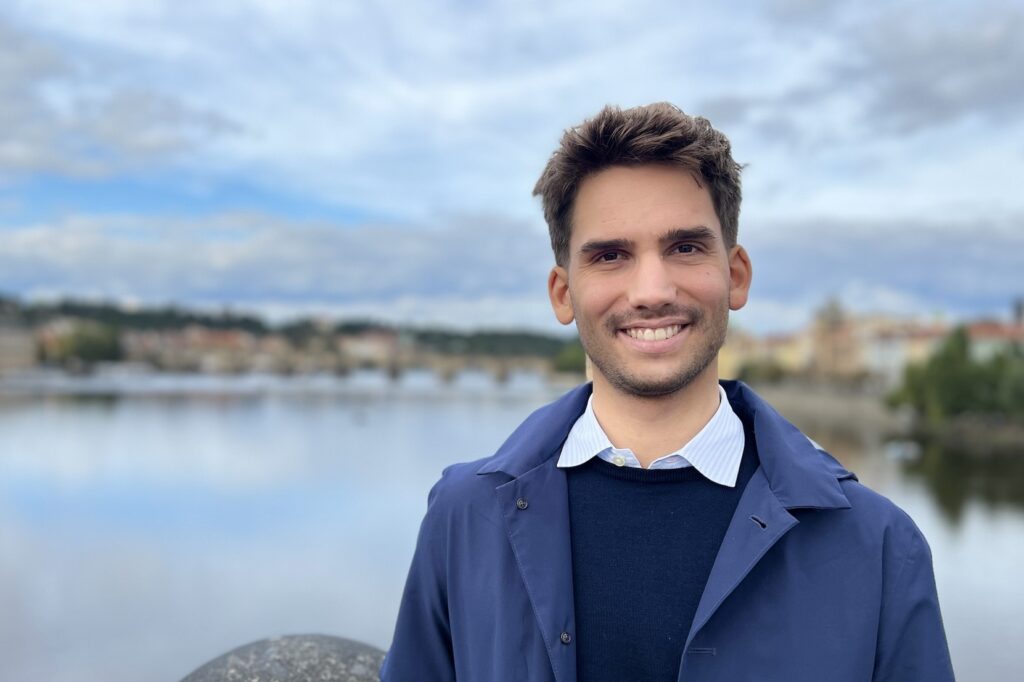
Andrea Ghezzi was born in Bergamo, Italy, in 1996. He obtained his Master degree cum laude in Automation and Control Engineering from Politecnico di Milano with a thesis about stochastic MPC with constraint prioritization. From December 2020 to September 2021 he was a researcher at Data Science R&D Department of Tenaris, in Dalmine, Italy, working on virtual sensing for improving the steelmaking process. From October 2021 he joined SYSCOP Laboratory at University of Freiburg, carrying out a PhD under the supervision of Prof. Dr. Moritz Diehl. He is a Marie-Curie fellow of the ELO-X project.
Project description
Andrea works mainly on algorithms for solving mixed-integer nonlinear problems (MINLPs), with a focus on problems arising from the transcription of optimal control problems via direct methods. Besides, he is interested in nonlinear model predictive control and its deployment on embedded hardware.
Publications
Dong, Shiying; Ghezzi, Andrea; Harzer, Jakob; Frey, Jonathan; Gao, Bingzhao; Chen, Hong; Diehl, Moritz
Real-Time NMPC With Convex--Concave Constraints and Application to Eco-Driving Journal Article
In: IEEE Transactions on Control Systems Technology, 2024.
@article{dong2024real,
title = {Real-Time NMPC With Convex--Concave Constraints and Application to Eco-Driving},
author = {Shiying Dong and Andrea Ghezzi and Jakob Harzer and Jonathan Frey and Bingzhao Gao and Hong Chen and Moritz Diehl},
doi = {10.1109/TCST.2024.3494993},
year = {2024},
date = {2024-11-15},
journal = {IEEE Transactions on Control Systems Technology},
abstract = {In this brief, we propose a novel real-time numerical algorithm for solving nonlinear model predictive control (NMPC) with convex–concave constraints, which arise in various practical applications. Instead of requiring full convergence for each problem at every sampling time, the proposed algorithm, called real-time iteration sequential convex programming (RTI-SCP), solves only one convex subproblem but iterates as the problem evolves. Compared with previous methods, the RTI-SCP adopts a more refined approach by linearizing only the concave components of the constraints. It retains and efficiently utilizes all the underlying convex structures, thereby transforming subproblems into structured forms that can be solved using the existing tools. In addition, to the best of our knowledge, the widely investigated eco-driving control strategy for autonomous vehicles is now formulated for the first time into a convex–concave programming problem with strong theoretical properties. Eventually, the experimental results demonstrate that the proposed strategy can improve computational efficiency and overall control performance, and it is suitable for real-time implementation.},
keywords = {},
pubstate = {published},
tppubtype = {article}
}
Reiter, Rudolf; Ghezzi, Andrea; Baumgärtner, Katrin; Hoffman, Jasper; McAllister, Robert D; Diehl, Moritz
AC4MPC: Actor-Critic Reinforcement Learning for Nonlinear Model Predictive Control Working paper
2024.
@workingpaper{reiter2024ac4mpc,
title = {AC4MPC: Actor-Critic Reinforcement Learning for Nonlinear Model Predictive Control},
author = {Rudolf Reiter and Andrea Ghezzi and Katrin Baumgärtner and Jasper Hoffman and Robert D McAllister and Moritz Diehl },
url = {https://doi.org/10.48550/arXiv.2406.03995},
year = {2024},
date = {2024-06-06},
abstract = {Ac{MPC} and ac{RL} are two powerful control strategies with, arguably, complementary advantages. In this work, we show how actor-critic ac{RL} techniques can be leveraged to improve the performance of ac{MPC}. The ac{RL} critic is used as an approximation of the optimal value function, and an actor roll-out provides an initial guess for primal variables of the ac{MPC}. A parallel control architecture is proposed where each ac{MPC} instance is solved twice for different initial guesses. Besides the actor roll-out initialization, a shifted initialization from the previous solution is used. Thereafter, the actor and the critic are again used to approximately evaluate the infinite horizon cost of these trajectories. The control actions from the lowest-cost trajectory are applied to the system at each time step. We establish that the proposed algorithm is guaranteed to outperform the original ac{RL} policy plus an error term that depends on the accuracy of the critic and decays with the horizon length of the ac{MPC} formulation. Moreover, we do not require globally optimal solutions for these guarantees to hold. The approach is demonstrated on an illustrative toy example and an ac{AD} overtaking scenario.},
keywords = {},
pubstate = {published},
tppubtype = {workingpaper}
}
Ghezzi, Andrea; Roy, Wim Van; Sager, Sebastian; Diehl, Moritz
A Sequential Benders-based Mixed-Integer Quadratic Programming Algorithm Working paper
2024.
@workingpaper{ghezzi2024sequential,
title = {A Sequential Benders-based Mixed-Integer Quadratic Programming Algorithm},
author = {Andrea Ghezzi and Wim Van Roy and Sebastian Sager and Moritz Diehl},
url = {https://doi.org/10.48550/arXiv.2404.11786},
year = {2024},
date = {2024-04-17},
abstract = {For continuous decision spaces, nonlinear programs (NLPs) can be efficiently solved via sequential quadratic programming (SQP) and, more generally, sequential convex programming (SCP). These algorithms linearize only the nonlinear equality constraints and keep the outer convex structure of the problem intact. The aim of the presented sequential mixed-integer quadratic programming (MIQP) algorithm for mixed-integer nonlinear problems (MINLPs) is to extend the SQP/SCP methodology to MINLPs and leverage the availability of efficient MIQP solvers. The algorithm employs a three-step method in each iterate: First, the MINLP is linearized at a given iterate. Second, an MIQP with its feasible set restricted to a specific region around the current linearization point is formulated and solved. Third, the integer variables obtained from the MIQP solution are fixed, and only an NLP in the continuous variables is solved. The outcome of the third step is compared to previous iterates, and the best iterate so far is used as a linearization point in the next iterate. Crucially, the objective values and derivatives from all previous iterates are used to formulate the polyhedral region in the second step. The linear inequalities that define the region build on concepts from generalized Benders' decomposition for MINLPs. Although the presented MINLP algorithm is a heuristic method without any global optimality guarantee, it converges to the exact integer solution when applied to convex MINLP with a linear outer structure. The conducted numerical experiments demonstrate that the proposed algorithm is competitive with other open-source solvers for MINLP. Finally, we solve two mixed-integer optimal control problems (MIOCPs) transcribed into MINLPs via direct methods, showing that the presented algorithm can effectively deal with nonlinear equality constraints, a major hurdle for generic MINLP solvers.},
keywords = {},
pubstate = {published},
tppubtype = {workingpaper}
}
Simpson, Léo; Ghezzi, Andrea; Asprion, Jonas; Diehl, Moritz
An Efficient Method for the Joint Estimation of System Parameters and Noise Covariances for Linear Time-Variant Systems Proceedings Article
In: 2023 Conference of Decision and Control (CDC) , pp. 4524-4529, IEEE, Singapore, Singapore, 2024, ISSN: 2576-2370.
@inproceedings{Simpson2023EMJE,
title = {An Efficient Method for the Joint Estimation of System Parameters and Noise Covariances for Linear Time-Variant Systems },
author = {Léo Simpson and Andrea Ghezzi and Jonas Asprion and Moritz Diehl},
url = {https://arxiv.org/abs/2211.12302},
doi = {10.1109/CDC49753.2023.10383686},
issn = {2576-2370},
year = {2024},
date = {2024-01-19},
urldate = {2024-01-19},
booktitle = {2023 Conference of Decision and Control (CDC) },
pages = {4524-4529},
publisher = {IEEE},
address = {Singapore, Singapore},
abstract = {We present an optimization-based method for the joint estimation of system parameters and noise covariances of linear time-variant systems. Given measured data, this method maximizes the likelihood of the parameters. We solve the optimization problem of interest via a novel structure-exploiting solver. We present the advantages of the proposed approach over commonly used methods in the framework of Moving Horizon Estimation. Finally, we show the performance of the method through numerical simulations on a realistic example of a thermal system. In this example, the method can successfully estimate the model parameters in a short computational time.},
keywords = {},
pubstate = {published},
tppubtype = {inproceedings}
}
Ghezzi, Andrea; Simpson, Léo; Bürger, Adrian; Zeile, Clemens; Sager, Sebastian; Diehl, Moritz
A Voronoi-Based Mixed-Integer Gauss-Newton Algorithm for MINLP Arising in Optimal Control Proceedings Article
In: 2023 European Control Conference (ECC), pp. 1-7, IEEE, Bucharest, Romania, 2023, ISBN: 978-3-907144-08-4.
@inproceedings{Ghezzi2023a,
title = { A Voronoi-Based Mixed-Integer Gauss-Newton Algorithm for MINLP Arising in Optimal Control},
author = {Andrea Ghezzi and Léo Simpson and Adrian Bürger and Clemens Zeile and Sebastian Sager and Moritz Diehl},
doi = {https://doi.org/10.23919/ECC57647.2023.10178130},
isbn = {978-3-907144-08-4},
year = {2023},
date = {2023-07-17},
urldate = {2023-07-17},
booktitle = {2023 European Control Conference (ECC)},
pages = {1-7},
publisher = {IEEE},
address = {Bucharest, Romania},
abstract = {We present a new algorithm for addressing nonconvex Mixed-Integer Nonlinear Programs (MINLPs) where the cost function is of nonlinear least squares form. We exploit this structure by leveraging a Gauss-Newton quadratic approximation of the original MINLP, leading to the formulation of a Mixed-Integer Quadratic Program (MIQP), which can be solved efficiently. The integer solution of the MIQP is used to fix the integer variables of the original MINLP, resulting in a standard Nonlinear Program. We introduce an iterative procedure to repeat the optimization of the two programs in order to improve the solution. To guide the iterations towards unexplored regions, we devise a strategy to partition the integer solution space based on Voronoi diagrams. Finally, we first illustrate the algorithm on a simple example of MINLP and then test it on an example of real-world complexity concerning the optimal control of an energy system. Here, the new algorithm outperforms state-of-the-art methods, finding a solution with a lower objective value, at the cost of requiring an increased runtime compared to other approximate methods.},
keywords = {},
pubstate = {published},
tppubtype = {inproceedings}
}
Ghezzi, Andrea; Messerer, Florian; Balocco, Jacopo; Manzoni, Vincenzo; Diehl, Moritz
An Implicit and Explicit Dual Model Predictive Control Formulation for a Steel Recycling Process Journal Article
In: European Journal of Control, pp. 100841, 2023, ISSN: 0947-3580.
@article{GHEZZI2023100841,
title = {An Implicit and Explicit Dual Model Predictive Control Formulation for a Steel Recycling Process},
author = {Andrea Ghezzi and Florian Messerer and Jacopo Balocco and Vincenzo Manzoni and Moritz Diehl},
url = {https://www.sciencedirect.com/science/article/pii/S0947358023000705},
doi = {https://doi.org/10.1016/j.ejcon.2023.100841},
issn = {0947-3580},
year = {2023},
date = {2023-06-14},
urldate = {2023-06-14},
journal = {European Journal of Control},
pages = {100841},
abstract = {We present a formulation for both implicit and explicit dual model predictive control for a steel recycling process. The process consists in the production of new steel by choosing a combination of several different steel scraps with unknown pollutant content. The pollutant content can only be measured after a scrap combination is molten, allowing for inference on the pollutants in the different scrap heaps. The production cost should be minimized while ensuring high quality of the product through constraining the maximum amount of pollutant. The dual control formulation allows to achieve the optimal explore-exploit trade-off between uncertainty reduction and cost minimization for the examined problem. Specifically, the dual effect is obtained by considering the dependence of the future pollutant uncertainties on the scrap selection in the predictions. The implicit formulation promotes uncertainty reduction indirectly via the impact of active constraints on the objective, while the explicit formulation adds a heuristic cost on uncertainty to encourage active exploration. We compare the formulations by numerical simulations of a simplified but representative industrial steel recycling process. The results demonstrate the superiority of the two dual formulations with respect to a robustified but non-dual formulation.},
keywords = {},
pubstate = {published},
tppubtype = {article}
}
Ghezzi, Andrea; Hoffman, Jasper; Frey, Jonathan; Boedecker, Joschka; Diehl, Moritz
Imitation Learning from Nonlinear MPC via the Exact Q-Loss and its Gauss-Newton Approximation Proceedings Article
In: 2023 Conference on Decision and Control (CDC), pp. 4766-4771, IEEE, Singapore, Singapore, 2023, ISBN: 979-8-3503-0124-3.
@inproceedings{Ghezzi2023b,
title = {Imitation Learning from Nonlinear MPC via the Exact Q-Loss and its Gauss-Newton Approximation},
author = {Andrea Ghezzi and Jasper Hoffman and Jonathan Frey and Joschka Boedecker and Moritz Diehl},
url = {https://doi.org/10.48550/arXiv.2304.01782},
doi = {10.1109/CDC49753.2023.10383323},
isbn = {979-8-3503-0124-3},
year = {2023},
date = {2023-01-19},
urldate = {2023-01-19},
booktitle = {2023 Conference on Decision and Control (CDC)},
pages = {4766-4771},
publisher = {IEEE},
address = {Singapore, Singapore},
abstract = {This work presents a novel loss function for learning nonlinear Model Predictive Control policies via Imitation Learning. Standard approaches to Imitation Learning neglect information about the expert and generally adopt a loss function based on the distance between expert and learned controls. In this work, we present a loss based on the Q-function directly embedding the performance objectives and constraint satisfaction of the associated Optimal Control Problem (OCP). However, training a Neural Network with the Q-loss requires solving the associated OCP for each new sample. To alleviate the computational burden, we derive a second Q-loss based on the Gauss-Newton approximation of the OCP resulting in a faster training time. We validate our losses against Behavioral Cloning, the standard approach to Imitation Learning, on the control of a nonlinear system with constraints. The final results show that the Q-function-based losses significantly reduce the amount of constraint violations while achieving comparable or better closed-loop costs.},
keywords = {},
pubstate = {published},
tppubtype = {inproceedings}
}