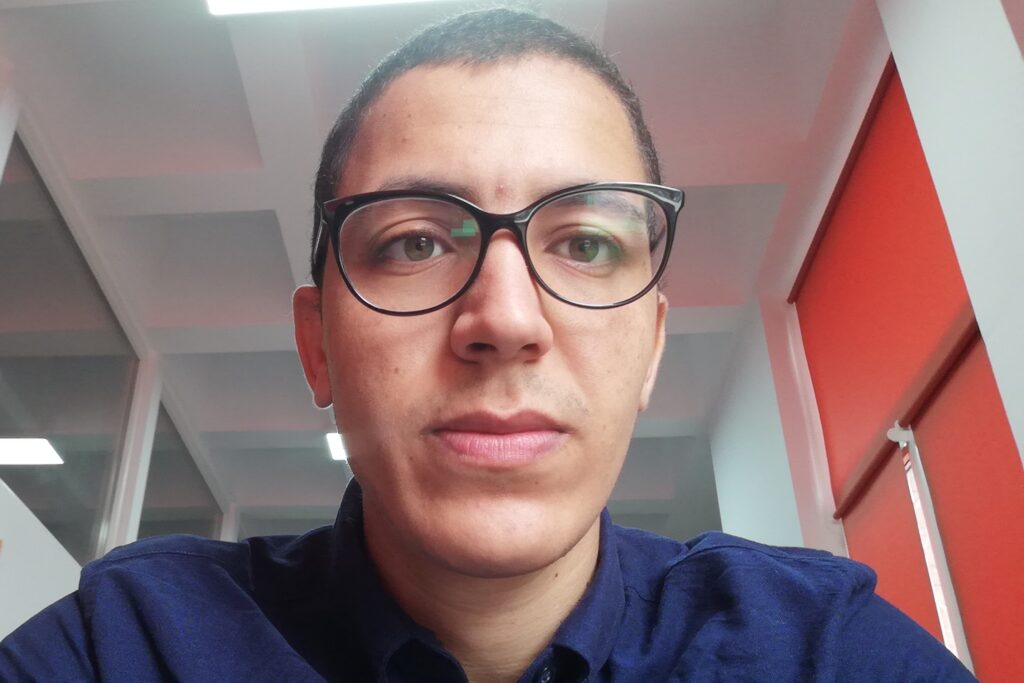
Lahcen El Bourkhissi, born in Morocco in 1997, earned his master’s degree in electrical engineering from the Arts et Métiers Institute of Technology in Lille, France, where he completed a thesis on the sensorless control of multi-phase permanent magnet synchronous machines using neural networks. In October 2021, he joined the University POLITEHNICA of Bucharest to pursue a PhD under the supervision of Prof. Dr. Ion Necoara as a Marie-Curie fellow in the ELO-X project.
Project description
Lahcen primarily focuses on developing penalty/augmented Lagrangian-based algorithms for solving nonlinear programs, which often arise from transcribing optimal control problems using direct methods. His main objective is to ensure that the developed methods involve only simple routines, making nonlinear model predictive control feasible for deployment on embedded hardware.
Publications
Bourkhissi, Lahcen El; Necoara, Ion
Convergence rates for an inexact linearized ADMM for nonsmooth optimization with nonlinear equality constraints Working paper
2024, (Under review).
@workingpaper{bourkhissi2024convergence,
title = {Convergence rates for an inexact linearized ADMM for nonsmooth optimization with nonlinear equality constraints},
author = {Lahcen El Bourkhissi and Ion Necoara},
year = {2024},
date = {2024-05-29},
urldate = {2024-05-29},
note = {Under review},
keywords = {},
pubstate = {published},
tppubtype = {workingpaper}
}
Bourkhissi, Lahcen El; Necoara, Ion
Complexity of linearized quadratic penalty for optimization with nonlinear equality constraints Working paper
2023, (Under review).
@workingpaper{bourkhissi2023complexity,
title = {Complexity of linearized quadratic penalty for optimization with nonlinear equality constraints},
author = {Lahcen El Bourkhissi and Ion Necoara},
doi = {https://doi.org/10.48550/arXiv.2402.15639},
year = {2023},
date = {2023-12-31},
abstract = {In this paper we consider a nonconvex optimization problem with nonlinear equality constraints. We assume that both, the objective function and the functional constraints, are locally smooth. For solving this problem, we propose a linearized quadratic penalty method, i.e., we linearize the objective function and the functional constraints in the penalty formulation at the current iterate and add a quadratic regularization, thus yielding a subproblem that is easy to solve, and whose solution is the next iterate. Under a dynamic regularization parameter choice, we derive convergence guarantees for the iterates of our method to an ϵ first-order optimal solution in O(1/ϵ3) outer iterations. Finally, we show that when the problem data satisfy Kurdyka-Lojasiewicz property, e.g., are semialgebraic, the whole sequence generated by our algorithm converges and we derive convergence rates. We validate the theory and the performance of the proposed algorithm by numerically comparing it with the existing methods from the literature.},
note = {Under review},
keywords = {},
pubstate = {published},
tppubtype = {workingpaper}
}
Bourkhissi, Lahcen El; Necoara, Ion; Patrinos, Panagiotis
Linearized ADMM for Nonsmooth Nonconvex Optimization with Nonlinear Equality Constraints Proceedings Article
In: 2023 62nd IEEE Conference on Decision and Control (CDC), pp. 7312-7317, IEEE, Singapore, Singapore, 2023, ISSN: 2576-2370.
@inproceedings{Lahcen23LinADMM,
title = {Linearized ADMM for Nonsmooth Nonconvex Optimization with Nonlinear Equality Constraints},
author = {Lahcen El Bourkhissi and Ion Necoara and Panagiotis Patrinos},
doi = {10.1109/CDC49753.2023.10384166},
issn = {2576-2370},
year = {2023},
date = {2023-12-13},
urldate = {2023-12-13},
booktitle = {2023 62nd IEEE Conference on Decision and Control (CDC)},
pages = {7312-7317},
publisher = {IEEE},
address = {Singapore, Singapore},
abstract = {This paper proposes a new approach for solving a structured nonsmooth nonconvex optimization problem with nonlinear equality constraints, where both the objective function and constraints are 2-blocks separable. Our method is based on a 2-block linearized ADMM, where we linearize the smooth part of the cost function and the nonlinear term of the functional constraints in the augmented Lagrangian at each outer iteration. This results in simple subproblems, whose solutions are used to update the iterates of the 2 blocks variables. We prove global convergence for the sequence generated by our method to a stationary point of the original problem. To demonstrate its effectiveness, we apply our proposed algorithm as a solver for the nonlinear model predictive control problem of an inverted pendulum on a cart.},
keywords = {},
pubstate = {published},
tppubtype = {inproceedings}
}
Ionescu, Tudor C.; Bourkhissi, Lahcen El; Necoara, Ion
Least Squares Moment Matching-Based Model Reduction Using Convex Optimization Proceedings Article
In: 2022 26th International Conference on System Theory, Control and Computing (ICSTCC), pp. 325–330, 2022, ISSN: 2372-1618.
@inproceedings{ionescuLeastSquaresMoment2022,
title = {Least Squares Moment Matching-Based Model Reduction Using Convex Optimization},
author = {Tudor C. Ionescu and Lahcen El Bourkhissi and Ion Necoara},
doi = {10.1109/ICSTCC55426.2022.9931837},
issn = {2372-1618},
year = {2022},
date = {2022-01-01},
booktitle = {2022 26th International Conference on System Theory, Control and Computing (ICSTCC)},
pages = {325--330},
keywords = {},
pubstate = {published},
tppubtype = {inproceedings}
}