PhD Candidate in Information Technology – Systems and Control
Dipartimento di Elettronica, Informazione e Bioingegneria (DEIB)
Politecnico di Milano
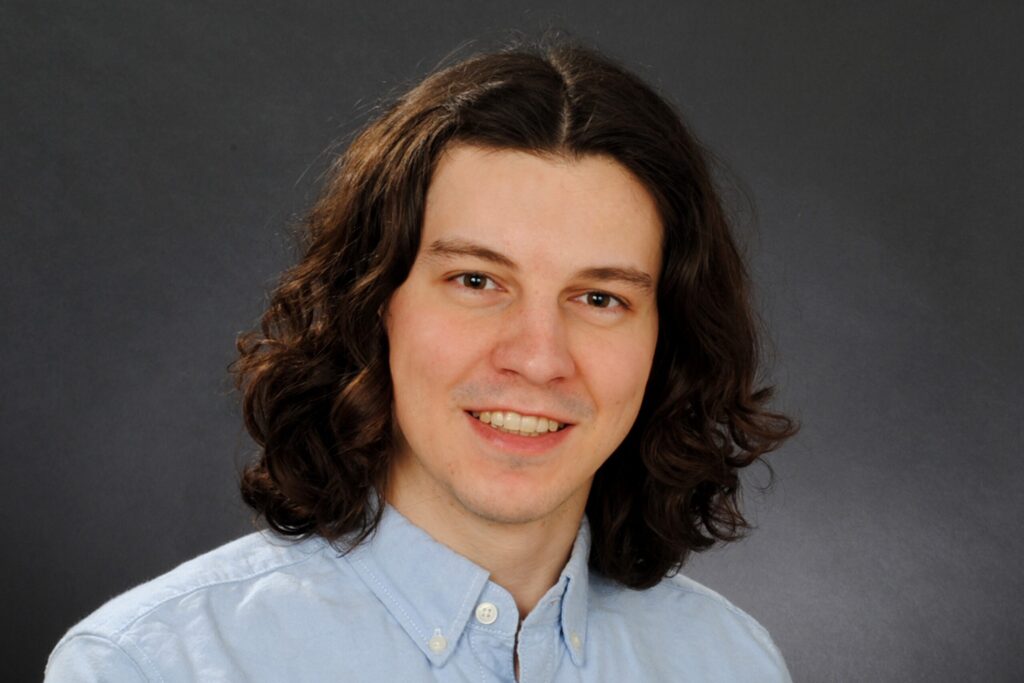
Nicolas Kessler graduated in mechatronics at Karlsruhe Institute of Technology in 2021 with focus on software engineering and control theory. Originally born in Germany, he also lived in France and China. During his studies he participated at Kamaro Engineering e. V. working on modular field robots. His Master thesis was on the modeling of the space maneuvering and docking vehicles at the test facility at University Würzburg.
Since May 2021 he is carrying out research activity at Politecnico di Milano, Italy, for ELO-X.
Project description
The next generation of highly automated, interconnected, and collaborative industrial systems will require solutions able to: (a) monitor in real-time the system and components’ behavior to estimate their conditions and spot possible faults or anomalies, and (b) manage many interacting subsystems and processes operating at different timescales, with a hierarchical topology, subject to constraints and performance requirements. These two tasks are strongly connected, since condition monitoring and fault detection must be fully integrated in the automation and control system. However, the current design approaches lack a systematic way to carry out such integration. To overcome this challenge, Nicolas will develop a design methodology for automation and control systems where learning-based solutions for condition monitoring, fault detection and recovery are implemented at multiple levels and time-scales in complex hierarchical control systems, and seamlessly integrated with optimization-based decision and control algorithms. The idea is to develop a high-level model predictive controller, running at slower frequency, that coordinates plant-wide operation and monitor its status using learning-based approaches. At lower layers, local controllers will exploit embedded learning and optimization to regulate single processes, monitor them and learn on-line their performance and conditions, implementing the directives received from the higher layers and sending them a feedback about the local status. Based on the overall feedback received from the lower levels, the high-level controller will readjust plant operation with the goal to maximize performance while guaranteeing safety and reliability.
Publications
Kessler, Nicolas; Fagiano, Lorenzo
On gain scheduling trajectory stabilization for nonlinear systems: theoretical insights and experimental results Journal Article
In: International Journal of Robust and Nonlinear Control, 2025.
@article{kessler2024gain,
title = {On gain scheduling trajectory stabilization for nonlinear systems: theoretical insights and experimental results},
author = {Nicolas Kessler and Lorenzo Fagiano },
url = {https://onlinelibrary.wiley.com/doi/full/10.1002/rnc.7784},
doi = {https://doi.org/10.1002/rnc.7784},
year = {2025},
date = {2025-01-07},
journal = {International Journal of Robust and Nonlinear Control},
abstract = {Steering a nonlinear system from an initial state to a desired one is a common task in control. While a nominal trajectory can be obtained rather systematically using a model, for example via numerical optimization, heuristics, or reinforcement learning, the design of a computationally fast and reliable feedback control law that guarantees rev{bounded deviations around} the found trajectory can be much more involved.
An approach that does not require high online computational power and is well-accepted in industry is gain-scheduling.
The results presented here pertain to the rev{boundedness} guarantees and rev{the set of safe initial conditions} of gain scheduled control laws, based on subsequent linearizations along the reference trajectory. The approach bounds the uncertainty arising from the linearization process, builds polytopic sets of linear time varying systems covering the nonlinear dynamics along the trajectory, and exploits sufficient conditions rev{for the existence of a robust polyquadratic Lyapunov function} to attempt the derivation of the desired gain-scheduled controller, via the solution of Linear Matrix Inequalities (LMIs). A result to estimate an ellipsoidal rev{set of safe initial conditions} is provided too. Moreover, arbitrary scheduling strategies between the control gains are considered in the analysis, and the method can be used also to check/assess the rev{boundedness} properties obtained with an existing gain-scheduled law.
The approach is demonstrated experimentally on a small quadcopter, as well as in simulation to design a scheduled controller for a chemical reactor model and to validate an existing control law for a gantry crane model.},
keywords = {},
pubstate = {published},
tppubtype = {article}
}
An approach that does not require high online computational power and is well-accepted in industry is gain-scheduling.
The results presented here pertain to the rev{boundedness} guarantees and rev{the set of safe initial conditions} of gain scheduled control laws, based on subsequent linearizations along the reference trajectory. The approach bounds the uncertainty arising from the linearization process, builds polytopic sets of linear time varying systems covering the nonlinear dynamics along the trajectory, and exploits sufficient conditions rev{for the existence of a robust polyquadratic Lyapunov function} to attempt the derivation of the desired gain-scheduled controller, via the solution of Linear Matrix Inequalities (LMIs). A result to estimate an ellipsoidal rev{set of safe initial conditions} is provided too. Moreover, arbitrary scheduling strategies between the control gains are considered in the analysis, and the method can be used also to check/assess the rev{boundedness} properties obtained with an existing gain-scheduled law.
The approach is demonstrated experimentally on a small quadcopter, as well as in simulation to design a scheduled controller for a chemical reactor model and to validate an existing control law for a gantry crane model.
Kessler, Nicolas; Fagiano, Lorenzo
On the design of terminal ingredients for linear time varying model predictive control: Theory and experimental application Proceedings Article
In: 8th IFAC Conference on Nonlinear Model Predictive Control NMPC 2024, pp. 263–268, IFAC-PapersOnLine, 2024, ISSN: 2405-8963.
@inproceedings{kessler2024design,
title = {On the design of terminal ingredients for linear time varying model predictive control: Theory and experimental application},
author = {Nicolas Kessler and Lorenzo Fagiano },
url = {https://www.sciencedirect.com/science/article/pii/S2405896324014204},
doi = {https://doi.org/10.1016/j.ifacol.2024.09.041},
issn = {2405-8963},
year = {2024},
date = {2024-09-25},
booktitle = {8th IFAC Conference on Nonlinear Model Predictive Control NMPC 2024},
number = {18},
issue = {58},
pages = {263--268},
publisher = {IFAC-PapersOnLine},
abstract = {The use of Linear Time Varying (LTV) Model Predictive Control (MPC) to stabilize a set of trajectories of a nonlinear system is considered. This technique has been successfully applied in simulations and experiments, but only few contributions investigate stability aspects and the essential involved quantities: the terminal penalty and terminal constraint. Deriving the former is not always thoroughly addressed or it is based on the -rather restrictive- assumption that the whole set of linearized dynamics is quadratically stabilizable. In this article, we propose Linear Matrix Inequality (LMI) conditions to co-design a gain-scheduled auxiliary feedback and Lyapunov function, used to derive offline terminal set conditions and a terminal penalty constraint for an LTV MPC scheme guaranteeing stability and recursive constraint satisfaction. Recent results by the authors are extended to the case of a varying stage cost, such that the controller can be tuned to meet time-varying trade-offs between tracking accuracy and input activity. The approach is demonstrated in embedded hardware running on a CrazyFlie drone.},
keywords = {},
pubstate = {published},
tppubtype = {inproceedings}
}
Kessler, Nicolas
Linear matrix inequality conditions for gain-scheduling and model predictive control PhD Thesis
2024.
@phdthesis{kessler2024phdthesis,
title = {Linear matrix inequality conditions for gain-scheduling and model predictive control},
author = {Nicolas Kessler},
url = {https://www.politesi.polimi.it/handle/10589/224812},
year = {2024},
date = {2024-09-17},
abstract = {This dissertation presents a novel approach to gain-scheduling model predictive control (MPC) for trajectory tracking on uncertain nonlinear systems, leveraging linear parameter-varying (LPV) models. A hierarchical scheme is developed, separating trajectory generation from stabilization using a 2-Degrees-of-Freedom (DoF) design. The focus of this thesis is the design of the feedback action, such that it guarantees tracking of the reference under bound satisfaction.
A key innovation is the graph-based gain-scheduling variable, enabling modular feedback application for online decisions. Nonlinearities are taken into account by extending the resulting LPV model with a polytopic uncertainty. Initially, a simple Linear Matrix Inequality (LMI) conditions are proposed to address stabilizability and later extended to address performance in an MPC scheme. Subsequently, it yields a novel method for the systematic design of the terminal ingredients for an LTV MPC. The LTV MPC is then extended to a robust tube-MPC with constraint satisfaction.
Efficient offline solvability of the resulting LMI conditions is addressed via the Alternating Direction Method of Multipliers (ADMM) to enable memory-efficient, distributed optimization.
The proposed LTV MPC scheme is computationally efficient online, because the optimal control problem is structured as a convex Quadratic Program (QP), that exploits its temporal evolution.
Simulation on a Continuously Stirred Tank Reactor (CSTR) and hardware implementation on a CrazyFlie drone demonstrate the approach's capability to stabilize nonlinear systems under disturbances and constraints with limited computing resources.
These advancements, combined with efficient offline LMI solving, promise broad applicability for safety-critical industrial systems.},
keywords = {},
pubstate = {published},
tppubtype = {phdthesis}
}
A key innovation is the graph-based gain-scheduling variable, enabling modular feedback application for online decisions. Nonlinearities are taken into account by extending the resulting LPV model with a polytopic uncertainty. Initially, a simple Linear Matrix Inequality (LMI) conditions are proposed to address stabilizability and later extended to address performance in an MPC scheme. Subsequently, it yields a novel method for the systematic design of the terminal ingredients for an LTV MPC. The LTV MPC is then extended to a robust tube-MPC with constraint satisfaction.
Efficient offline solvability of the resulting LMI conditions is addressed via the Alternating Direction Method of Multipliers (ADMM) to enable memory-efficient, distributed optimization.
The proposed LTV MPC scheme is computationally efficient online, because the optimal control problem is structured as a convex Quadratic Program (QP), that exploits its temporal evolution.
Simulation on a Continuously Stirred Tank Reactor (CSTR) and hardware implementation on a CrazyFlie drone demonstrate the approach's capability to stabilize nonlinear systems under disturbances and constraints with limited computing resources.
These advancements, combined with efficient offline LMI solving, promise broad applicability for safety-critical industrial systems.
Kessler, Nicolas; Fagiano, Lorenzo
On the Design of Linear Time Varying Model Predictive Control for Trajectory Stabilization Working paper
2024.
@workingpaper{kessler2024lticontrol,
title = { On the Design of Linear Time Varying Model Predictive Control for Trajectory Stabilization},
author = {Nicolas Kessler and Lorenzo Fagiano },
year = {2024},
date = {2024-01-01},
abstract = {Stabilizing a reference trajectory of a nonlinear system is a recurrent, non-trivial task in control engineering. A common approach is to linearize the dynamics along the trajectory, thus deriving a linear-time-varying (LTV) model, and to design a model predictive controller (MPC), which results to be computationally efficient, since only convex programs need to be solved in real time, while retaining constraint handling capabilities. Building on recent developments in gain-scheduling control design,
where linearization errors and tracking error bounds are considered, a new approach to derive such LTV-MPC controllers is presented. The method addresses the systematic derivation of a suitable terminal cost. The resulting MPC law is tube-based, exploiting the co-designed auxiliary gain-scheduled controller.
Computational and implementation aspects are discussed as well, and the resulting hierarchical method is demonstrated both in simulation and in experiments with a small drone with fast dynamics and limited embedded computational capacity.},
keywords = {},
pubstate = {published},
tppubtype = {workingpaper}
}
where linearization errors and tracking error bounds are considered, a new approach to derive such LTV-MPC controllers is presented. The method addresses the systematic derivation of a suitable terminal cost. The resulting MPC law is tube-based, exploiting the co-designed auxiliary gain-scheduled controller.
Computational and implementation aspects are discussed as well, and the resulting hierarchical method is demonstrated both in simulation and in experiments with a small drone with fast dynamics and limited embedded computational capacity.
Kessler, Nicolas; Fagiano, Lorenzo
On the stabilization of forking and cyclic trajectories for nonlinear systems Proceedings Article
In: 3rd Modeling, Estimation and Control Conference MECC 2023, pp. 199–204, IFAC-PapersOnLine, Lake Tahoe, NV, USA, 2023, ISSN: 2405-8963.
@inproceedings{kessler2023stabilization,
title = {On the stabilization of forking and cyclic trajectories for nonlinear systems},
author = {Nicolas Kessler and Lorenzo Fagiano },
url = {https://www.sciencedirect.com/science/article/pii/S2405896323023571},
doi = {https://doi.org/10.1016/j.ifacol.2023.12.024},
issn = {2405-8963},
year = {2023},
date = {2023-12-03},
urldate = {2023-12-03},
booktitle = {3rd Modeling, Estimation and Control Conference MECC 2023},
volume = {56},
number = {3},
pages = {199--204},
publisher = {IFAC-PapersOnLine},
address = {Lake Tahoe, NV, USA},
abstract = {Stabilizing a reference trajectory for a nonlinear system is a common, non-trivial task in control theory. An approach to solve this problem is to approximate the nonlinear system along the trajectory as an uncertain linear time-varying one, and to solve an optimization problem featuring Linear Matrix Inequality (LMI) constraints to derive a stabilizing, smooth, gain-scheduled control law. Such an approach is extended here by considering a set of reference trajectories instead of a single one, such that switching among them is permitted. These switching events are commonly encountered in industrial plants, such as energy generation systems, and are of high relevance in practice. The approach allows one to derive a gain-scheduled control law guaranteeing asymptotic stability also during the switching and accounting for the linearization errors. Simulation results on a chemical system highlight the effectiveness of the method.},
keywords = {},
pubstate = {published},
tppubtype = {inproceedings}
}
Kessler, Nicolas; Fagiano, Lorenzo
On Control of Phase Transitions in Airborne Wind Energy Systems Workshop
2022.
@workshop{kessler2022control,
title = {On Control of Phase Transitions in Airborne Wind Energy Systems},
author = {Nicolas Kessler and Lorenzo Fagiano},
url = {https://repository.tudelft.nl/file/File_be6082e6-7052-44c7-ae2a-9e2597f291cf},
year = {2022},
date = {2022-01-01},
urldate = {2022-01-01},
keywords = {},
pubstate = {published},
tppubtype = {workshop}
}