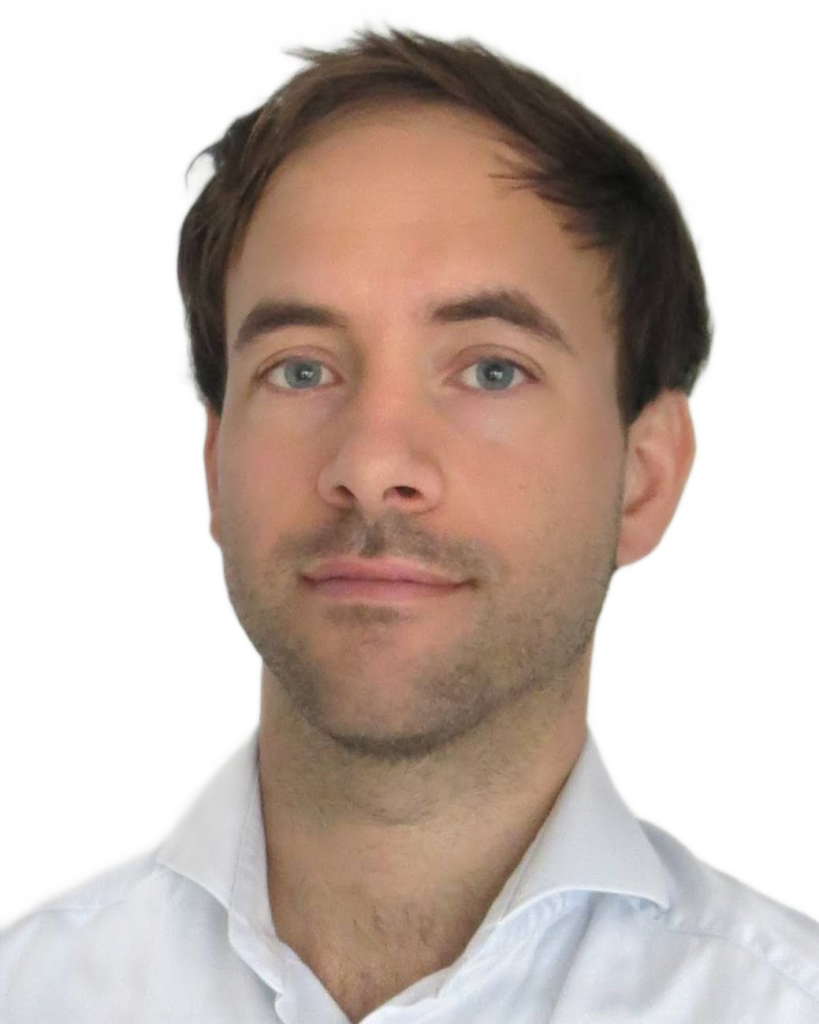
Rudolf Reiter graduated 2016 with a Master’s degree in electrical engineering with a focus on control systems from the Graz University of Technology in Austria. During that time he did internships at Bernecker & Rainer (ABB) and an exchange semester at the University of Utah, USA. Afterwards, he joined an international company for measurement devices (Anton Paar GmbH) where he worked as a specialist for control systems and signal processing. In 2018 he started working as a researcher for autonomous driving at a vehicle research company. There he also joined the Autonomous Racing Graz team, which competes at real-world autonomous racing events.
Project description
Rudolf Reiter is actively engaged in cutting-edge research in the field of autonomous driving and autonomous racing as part of his pursuit of a Ph.D. His work primarily focuses on several key areas, including model predictive control, mixed integer optimization, optimal control, and reinforcement learning. By combining expertise in these domains, he aims to develop innovative solutions that can enhance the performance and safety of autonomous vehicles, especially in strategic racing scenarios and combinatorial sophisticated multi-lane traffic. His research promises to contribute significantly to the advancement of autonomous systems, making them more efficient and capable in navigating complex environments while pushing the boundaries of autonomous racing technology.
Publications
Reiter, Rudolf; Nurkanovic, Armin; Frey, Jonathan; Diehl, Moritz
Frenet-Cartesian model representations for automotive obstacle avoidance within nonlinear MPC Journal Article
In: European Journal of Control, vol. 74, pp. 100847, 2023, ISSN: 0947-3580, (2023 European Control Conference Special Issue).
@article{REITER2023100847,
title = {Frenet-Cartesian model representations for automotive obstacle avoidance within nonlinear MPC},
author = {Rudolf Reiter and Armin Nurkanovic and Jonathan Frey and Moritz Diehl},
url = {https://www.sciencedirect.com/science/article/pii/S0947358023000766},
doi = {https://doi.org/10.1016/j.ejcon.2023.100847},
issn = {0947-3580},
year = {2023},
date = {2023-11-01},
journal = {European Journal of Control},
volume = {74},
pages = {100847},
abstract = {In recent years, nonlinear model predictive control has been extensively used for solving automotive motion control and planning tasks. In order to formulate the nonlinear model predictive control problem, different coordinate systems can be used with different advantages. We propose and compare formulations for the nonlinear MPC related optimization problem, involving a Cartesian and a Frenet coordinate frame in a single nonlinear program. We specify costs and collision avoidance constraints in the more advantageous coordinate frame, derive appropriate formulations and compare different obstacle constraints. With this approach, we exploit the simpler formulation of opponent vehicle constraints in the Cartesian coordinate frame, as well as road-aligned costs and constraints related to the Frenet coordinate frame. Comparisons to other approaches in a simulation framework highlight the advantages of the proposed methods.},
note = {2023 European Control Conference Special Issue},
keywords = {},
pubstate = {published},
tppubtype = {article}
}
Mamedov, Shamil; Reiter, Rudolf; Azad, Seyed Mahdi Basiri; Boedecker, Joschka; Diehl, Moritz; Swevers, Jan
Safe Imitation Learning of Nonlinear Model Predictive Control for Flexible Robots Working paper
2023, (Submitted to ICRA 2024).
@workingpaper{mamedov2023safe,
title = {Safe Imitation Learning of Nonlinear Model Predictive Control for Flexible Robots},
author = {Shamil Mamedov and Rudolf Reiter and Seyed Mahdi Basiri Azad and Joschka Boedecker and Moritz Diehl and Jan Swevers},
url = {https://doi.org/10.48550/arXiv.2212.02941},
year = {2023},
date = {2023-09-28},
abstract = {Flexible robots may overcome some of the industry's major challenges, such as enabling intrinsically safe human-robot collaboration and achieving a higher load-to-mass ratio. However, controlling flexible robots is complicated due to their complex dynamics, which include oscillatory behavior and a high-dimensional state space. NMPC offers an effective means to control such robots, but its extensive computational demands often limit its application in real-time scenarios. To enable fast control of flexible robots, we propose a framework for a safe approximation of NMPC using imitation learning and a predictive safety filter. Our framework significantly reduces computation time while incurring a slight loss in performance. Compared to NMPC, our framework shows more than a eightfold improvement in computation time when controlling a three-dimensional flexible robot arm in simulation, all while guaranteeing safety constraints. Notably, our approach outperforms conventional reinforcement learning methods. The development of fast and safe approximate NMPC holds the potential to accelerate the adoption of flexible robots in industry.},
note = {Submitted to ICRA 2024},
keywords = {},
pubstate = {published},
tppubtype = {workingpaper}
}
Reiter, Rudolf; Hoffman, Jasper; Boedecker, Joschka; Diehl, Moritz
A Hierarchical Approach for Strategic Motion Planning in Autonomous Racing Proceedings Article
In: 2023 European Control Conference (ECC), pp. 1-8, IEEE, Bucharest, Romania, 2023, ISBN: 978-3-907144-08-4.
@inproceedings{Reiter2023Hierarchical,
title = {A Hierarchical Approach for Strategic Motion Planning in Autonomous Racing},
author = {Rudolf Reiter and Jasper Hoffman and Joschka Boedecker and Moritz Diehl},
doi = {10.23919/ECC57647.2023.10178143},
isbn = {978-3-907144-08-4},
year = {2023},
date = {2023-07-17},
urldate = {2023-07-17},
booktitle = {2023 European Control Conference (ECC)},
pages = {1-8},
publisher = {IEEE},
address = {Bucharest, Romania},
abstract = {We present an approach for safe trajectory planning, where a strategic task related to autonomous racing is learned sample efficiently within a simulation environment. A high-level policy, represented as a neural network, outputs a reward specification that is used within the function of a parametric nonlinear model predictive controller. By including constraints and vehicle kinematics in the nonlinear program, we can guarantee safe and feasible trajectories related to the used model. Compared to classical reinforcement learning, our approach restricts the exploration to safe trajectories, starts with an excellent prior performance and yields complete trajectories that can be passed to a tracking lowest-level controller. We do not address the lowest-level controller in this work and assume perfect tracking of feasible trajectories. We show the superior performance of our algorithm on simulated racing tasks that include high-level decision-making. The vehicle learns to efficiently overtake slower vehicles and avoids getting overtaken by blocking faster ones.},
keywords = {},
pubstate = {published},
tppubtype = {inproceedings}
}
Baumgärtner, Katrin; Reiter, Rudolf; Diehl, Moritz
Moving Horizon Estimation with Adaptive Regularization for Ill-Posed State and Parameter Estimation Problems Proceedings Article
In: 2022 IEEE 61st Conference on Decision and Control (CDC), pp. 2165-2171, IEEE, Cancun, Mexico, 2023, ISBN: 978-1-6654-6761-2.
@inproceedings{Baumgärtner2022MHE,
title = {Moving Horizon Estimation with Adaptive Regularization for Ill-Posed State and Parameter Estimation Problems},
author = {Katrin Baumgärtner and Rudolf Reiter and Moritz Diehl},
doi = {10.1109/CDC51059.2022.9993416},
isbn = {978-1-6654-6761-2},
year = {2023},
date = {2023-01-10},
urldate = {2023-01-10},
booktitle = {2022 IEEE 61st Conference on Decision and Control (CDC)},
pages = {2165-2171},
publisher = {IEEE},
address = {Cancun, Mexico},
abstract = {We investigate the usage of Moving Horizon Estimation (MHE) for state and parameter estimation for partially non-detectable systems with measurements corrupted by outliers. We propose an arrival cost update formula based on the Generalized Gauss-Newton method and illustrate how it can be generalized to nonconvex loss functions that can be effectively used for outlier rejection. Moreover, we propose an adaptive regularization scheme for the arrival cost which introduces forgetting as well as additional pseudo-measurements to the arrival cost update. We illustrate the performance of the proposed algorithms on a longitudinal vehicle state and parameter estimation problem.},
keywords = {},
pubstate = {published},
tppubtype = {inproceedings}
}
Reiter, Rudolf; Messerer, Florian; Schratter, Markus; Watzenig, Daniel; Diehl, Moritz
An Inverse Optimal Control Approach for Trajectory Prediction of Autonomous Race Cars Proceedings Article
In: 2022 European Control Conference (ECC), pp. 146–153, IEEE, London, United Kingdom, 2022, ISBN: 978-3-907144-07-7.
@inproceedings{reiter_inverse_2022,
title = {An Inverse Optimal Control Approach for Trajectory Prediction of Autonomous Race Cars},
author = {Rudolf Reiter and Florian Messerer and Markus Schratter and Daniel Watzenig and Moritz Diehl},
url = {https://ieeexplore.ieee.org/document/9838100/},
doi = {10.23919/ECC55457.2022.9838100},
isbn = {978-3-907144-07-7},
year = {2022},
date = {2022-07-01},
urldate = {2022-09-09},
booktitle = {2022 European Control Conference (ECC)},
pages = {146--153},
publisher = {IEEE},
address = {London, United Kingdom},
abstract = {This paper proposes an optimization-based approach to predict trajectories of autonomous race cars. We assume that the observed trajectory is the result of an optimization problem that trades off path progress against acceleration and jerk smoothness, and which is restricted by constraints. The algorithm predicts a trajectory by solving a parameterized nonlinear program (NLP) which contains path progress and smoothness in cost terms. By observing the actual motion of a vehicle, the parameters of prediction are updated by means of solving an inverse optimal control problem that contains the parameters of the predicting NLP as optimization variables. The algorithm therefore learns to predict the observed vehicle trajectory in a least-squares relation to measurement data and to the presumed structure of the predicting NLP. This work contributes with an algorithm that allows for accurate and interpretable predictions with sparse data. The algorithm is implemented on embedded hardware in an autonomous real-world race car that is competing in the challenge Roborace and analyzed with respect to recorded data.},
keywords = {},
pubstate = {published},
tppubtype = {inproceedings}
}