Learning-enhanced Model Predictive Control for Autonomous Driving
Rudolf Reiter, SYSCOP Laboratory, University of Freiburg
Nonlinear model predictive control is a well established technology for local trajectory planning and control in autonomous driving applications. Increased complexity occurs in racing scenarios that involve strategic decision making or multi-lane traffic scenarios, which involve combinatorial choices, such as lane changes or overtaking decisions. Derivative-based local optimization fails in this scenarios. Therefore, learning-enhanced formulations combined with mixed integer optimizations are introduced and examined as a promising technology with an advanced performance.
The work can be clustered in three main fields:
- Numerical efficient formulations for Nonlinear Model Predictive Control in autonomous driving.
- Dealing with the combinatorial complexity by either an efficient formulation or using novel neural network architectures that enhances the algorithm of combinatorial mixed-integer solvers.
- Dealing with the complexity of strategic decision making by including reinforcement learning as a high level decision maker and inverse optimal control as state estimator.
Numerical efficient formulations for Nonlinear Model Predictive Control in autonomous driving
As an essential basis of this work, an efficient nonlinear model predictive controller formulation is required. The work focuses on the representation in a curvilienar Frenet coordiante frame and the representation of moving obstacles in order to achieve high performance applications.
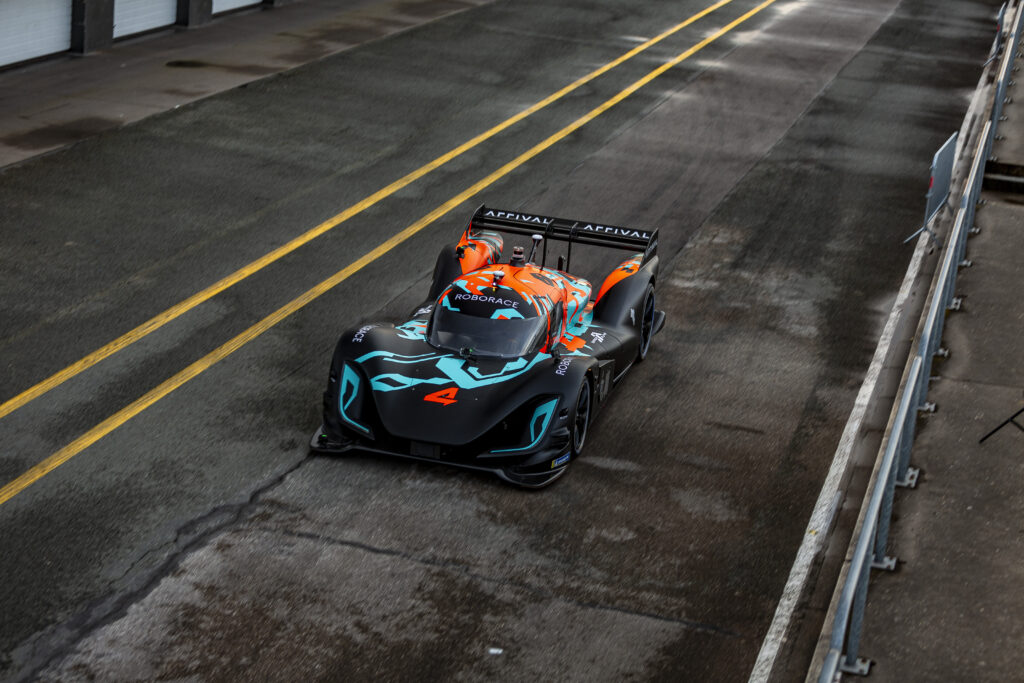
Efficient Mixed-Integer Formulations and Machine Learning for Combinatorial Optimization
Coming soon…
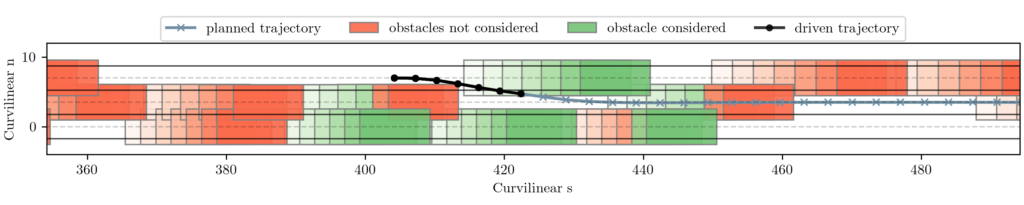
Strategic Decision Making by Inverse Optimal Control and Reinforcement Learning
Coming soon…
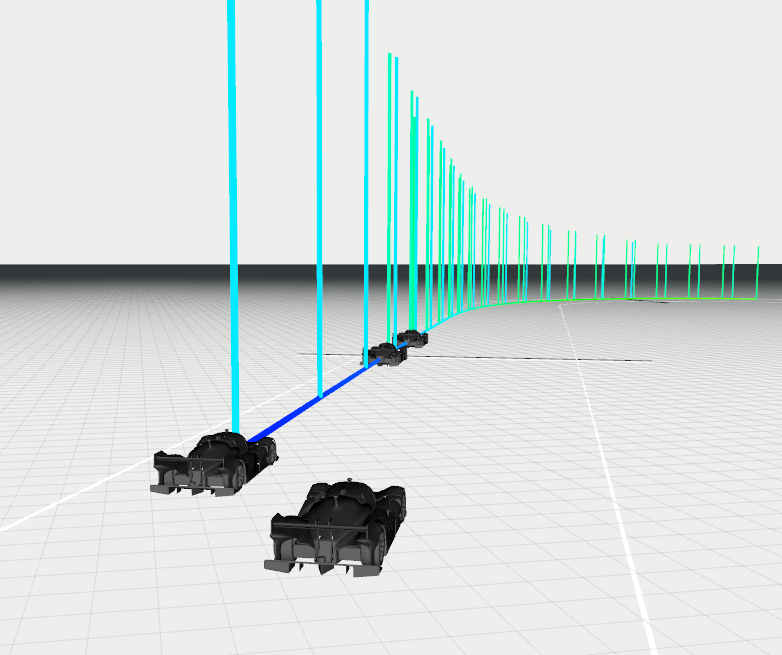