Florian Messerer
PhD Candidate
University of Freiburg
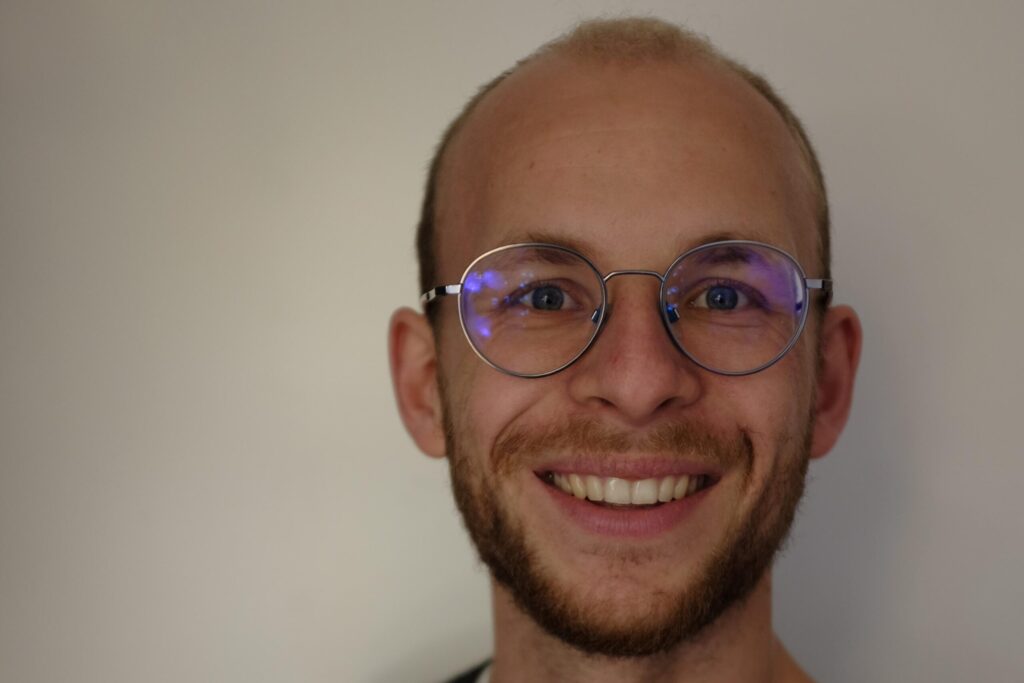
Florian Messerer received a B.Sc. in Microsystems Engineering from the University of Freiburg, Germany, in 2016 and a M.Sc. in Mathematical Engineering from KU Leuven, Belgium, in 2018. He then came back to Freiburg to join the Laboratory for Systems, Control and Optimization as research and teaching assistant. Since March 2020, he is pursuing a PhD under the supervision of Prof. Moritz Diehl.
Project description
While standard (nominal) model predictive control can react to disturbances, it has no explicit model of uncertainty. This is not sufficient to ensure robust constraint satisfaction, as a trajectory at the boundary of the feasible set can be tipped into infeasibility by a small perturbation. There are two main paradigms for uncertainty-aware MPC: stochastic MPC (SMPC) and robust MPC (RMPC). In both approaches, knowledge of the uncertainty is included in the system model and the optimal control problem (OCP) formulation. The specific challenges of both approaches are closely related: (a) the propagation of uncertainty sets through time, (b) fulfilling constraints with respect to these uncertainty sets and (c) the optimization over feedback policies to avoid the prediction of unreallistically fast growing uncertainty sets. By surmounting these challenges, one can ensure that an appropriate back-off is kept from constraints without the need for hand-engineered and heuristically chosen safety margins.
However, in their standard forms both RMPC and SMPC only consider static uncertainty estimates: they are not aware that uncertainty may be reduced by learning about the system. This is the topic of the field of dual control: how can the conflicting objectives of exploration to learn about the system dynamics and exploitation of the obtained knowledge be balanced? And more specifically: how can this trade-off be captured by the optimal control problem formulation, such that the trade-off is automatically optimally resolved without the need for heuristic problem modifications?
The focus of my PhD is on both efficient formulations and algorithms for uncertainty-aware (stochastic, robust, dual) optimal control and model predictive control. These two aims go hand in hand, as exploitation of the specific structure of a formulation is the basis for tailored algorithms.
Publications
Gao, Yunfan; Messerer, Florian; van Duijkeren, Niels; Houska, Boris; Diehl, Moritz
Real-Time-Feasible Collision-Free Motion Planning For Ellipsoidal Objects Proceedings Article Forthcoming
In: Forthcoming, (Accepted at the 2024 Conference on Decision and Control (CDC)).
@inproceedings{24_gao_realtimefeasible,
title = {Real-Time-Feasible Collision-Free Motion Planning For Ellipsoidal Objects},
author = {Yunfan Gao and Florian Messerer and Niels van Duijkeren and Boris Houska and Moritz Diehl},
doi = {https://doi.org/10.48550/arXiv.2409.12007},
year = {2024},
date = {2024-09-18},
abstract = {Online planning of collision-free trajectories is a fundamental task for robotics and self-driving car applications. This paper revisits collision avoidance between ellipsoidal objects using differentiable constraints. Two ellipsoids do not overlap if and only if the endpoint of the vector between the center points of the ellipsoids does not lie in the interior of the Minkowski sum of the ellipsoids. This condition is formulated using a parametric over-approximation of the Minkowski sum, which can be made tight in any given direction. The resulting collision avoidance constraint is included in an optimal control problem (OCP) and evaluated in comparison to the separating-hyperplane approach. Not only do we observe that the Minkowski-sum formulation is computationally more efficient in our experiments, but also that using pre-determined over-approximation parameters based on warm-start trajectories leads to a very limited increase in suboptimality. This gives rise to a novel real-time scheme for collision-free motion planning with model predictive control (MPC). Both the real-time feasibility and the effectiveness of the constraint formulation are demonstrated in challenging real-world experiments.},
note = {Accepted at the 2024 Conference on Decision and Control (CDC)},
keywords = {},
pubstate = {forthcoming},
tppubtype = {inproceedings}
}
Gao, Yunfan; Messerer, Florian; van Duijkeren, Niels; Diehl, Moritz
Stochastic Model Predictive Control with Optimal Linear Feedback for Mobile Robots in Dynamic Environments Proceedings Article
In: 8th IFAC Conference on Nonlinear Model Predictive Control NMPC 2024, pp. 153-158, IFAC-PapersOnLine, 2024.
@inproceedings{24_gao_stochasticmpc,
title = {Stochastic Model Predictive Control with Optimal Linear Feedback for Mobile Robots in Dynamic Environments},
author = {Yunfan Gao and Florian Messerer and Niels van Duijkeren and Moritz Diehl},
doi = {https://doi.org/10.1016/j.ifacol.2024.09.024},
year = {2024},
date = {2024-09-03},
urldate = {2024-09-03},
booktitle = {8th IFAC Conference on Nonlinear Model Predictive Control NMPC 2024},
volume = {58},
number = {18},
pages = {153-158},
publisher = {IFAC-PapersOnLine},
abstract = {Robot navigation around humans can be a challenging problem since human movements are hard to predict. Stochastic model predictive control (MPC) can account for such uncertainties and approximately bound the probability of a collision to take place. In this paper, to counteract the rapidly growing human motion uncertainty over time, we incorporate state feedback in the stochastic MPC. This allows the robot to more closely track reference trajectories. To this end the feedback policy is left as a degree of freedom in the optimal control problem. The stochastic MPC with feedback is validated in simulation experiments and is compared against nominal MPC and stochastic MPC without feedback. The added computation time can be limited by reducing the number of additional variables for the feedback law with a small compromise in control performance.},
keywords = {},
pubstate = {published},
tppubtype = {inproceedings}
}
Leeman, Antoine P.; Köhler, Johannes; Messerer, Florian; Lahr, Amon; Diehl, Moritz; Zeilinger, Melanie N.
Fast System Level Synthesis: Robust Model Predictive Control Using Riccati Recursions Proceedings Article
In: 8th IFAC Conference on Nonlinear Model Predictive Control NMPC 2024, IFAC-PapersOnLine, 2024.
@inproceedings{leeman_fast_2024,
title = {Fast System Level Synthesis: Robust Model Predictive Control Using Riccati Recursions},
author = {Antoine P. Leeman and Johannes Köhler and Florian Messerer and Amon Lahr and Moritz Diehl and Melanie N. Zeilinger},
url = {https://doi.org/10.48550/arXiv.2401.13762},
doi = {10.1016/j.ifacol.2024.09.027},
year = {2024},
date = {2024-09-01},
urldate = {2024-02-07},
booktitle = {8th IFAC Conference on Nonlinear Model Predictive Control NMPC 2024},
volume = {58},
number = {18},
publisher = {IFAC-PapersOnLine},
abstract = {System Level Synthesis (SLS) enables improved robust MPC formulations by allowing for joint optimization of the nominal trajectory and controller. This paper introduces a tailored algorithm for solving the corresponding disturbance feedback optimization problem. The proposed algorithm builds on a recently proposed joint optimization scheme and iterates between optimizing the controller and the nominal trajectory while converging q-linearly to an optimal solution. We show that the controller optimization can be solved through Riccati recursions leading to a horizon-length, state, and input scalability of O(N2(n3x+n3u)) for each iterate. On a numerical example, the proposed algorithm exhibits computational speedups of order 10 to 10^3 compared to general-purpose commercial solvers.
},
keywords = {},
pubstate = {published},
tppubtype = {inproceedings}
}
Messerer, Florian; Baumgärtner, Katrin; Nurkanovic, Armin; Diehl, Moritz
Approximate propagation of normal distributions for stochastic optimal control of nonsmooth systems Journal Article
In: Nonlinear Analysis: Hybrid Systems, vol. 53, pp. 101499, 2024, ISSN: 1751-570X.
@article{MESSERER2024101499,
title = {Approximate propagation of normal distributions for stochastic optimal control of nonsmooth systems},
author = {Florian Messerer and Katrin Baumgärtner and Armin Nurkanovic and Moritz Diehl},
url = {https://www.sciencedirect.com/science/article/pii/S1751570X24000360},
doi = {https://doi.org/10.1016/j.nahs.2024.101499},
issn = {1751-570X},
year = {2024},
date = {2024-08-01},
urldate = {2023-08-14},
journal = {Nonlinear Analysis: Hybrid Systems},
volume = {53},
pages = {101499},
abstract = {We present a method for the approximate propagation of mean and covariance of a probability distribution through ordinary differential equations (ODE) with discontinuous right-hand side. For piecewise affine systems, a normalization of the propagated probability distribution at every time step allows us to analytically compute the expectation integrals of the mean and covariance dynamics while explicitly taking into account the discontinuity. This leads to a natural smoothing of the discontinuity such that for relevant levels of uncertainty the resulting ODE can be integrated directly with standard schemes and it is neither necessary to prespecify the switching sequence nor to use a switch detection method. We then show how this result can be employed in the more general case of piecewise smooth functions based on a structure preserving linearization scheme. The resulting dynamics can be straightforwardly used within standard formulations of stochastic optimal control problems with chance constraints.},
keywords = {},
pubstate = {published},
tppubtype = {article}
}
Frey, Jonathan; Gao, Yunfan; Messerer, Florian; Lahr, Amon; Zeilinger, Melanie N.; Diehl, Moritz
Efficient Zero-Order Robust Optimization for Real-Time Model Predictive Control with Acados Proceedings Article
In: 2024 European Control Conference (ECC), IEEE, Stockholm, Sweden, 2024, ISBN: 978-3-9071-4410-7.
@inproceedings{frey_efficient_2023,
title = {Efficient Zero-Order Robust Optimization for Real-Time Model Predictive Control with Acados},
author = {Jonathan Frey and Yunfan Gao and Florian Messerer and Amon Lahr and Melanie N. Zeilinger and Moritz Diehl},
doi = {10.23919/ECC64448.2024.10591148},
isbn = {978-3-9071-4410-7},
year = {2024},
date = {2024-06-03},
urldate = {2023-12-18},
booktitle = {2024 European Control Conference (ECC)},
publisher = {IEEE},
address = {Stockholm, Sweden},
abstract = {Robust and stochastic optimal control problem (OCP) formulations allow a systematic treatment of uncertainty, but are typically associated with a high computational cost. The recently proposed zero-order robust optimization (zoRO) algorithm mitigates the computational cost of uncertainty-aware MPC by propagating the uncertainties separately from the nominal dynamics. This paper details the combination of zoRO with the real-time iteration (RTI) scheme and presents an efficient open-source implementation in acados, utilizing BLASFEO for the linear algebra operations. In addition to the scaling advantages posed by the zoRO algorithm, the efficient implementation drastically reduces the computational overhead, and, combined with an RTI scheme, enables the use of tube-based MPC for a wider range of applications. The flexibility, usability and effectiveness of the proposed implementation is demonstrated on two examples. On the practical example of a differential drive robot, the proposed implementation results in a tenfold reduction of computation time with respect to the previously available zoRO implementation.},
keywords = {},
pubstate = {published},
tppubtype = {inproceedings}
}
Messerer, Florian; Baumgärtner, Katrin; Lucia, Sergio; Diehl, Moritz
Fourth-order suboptimality of nominal model predictive control in the presence of uncertainty Journal Article
In: IEEE Control Systems Letters, vol. 8, pp. 508-513, 2024.
@article{messerer2024fourthorder,
title = {Fourth-order suboptimality of nominal model predictive control in the presence of uncertainty},
author = {Florian Messerer and Katrin Baumgärtner and Sergio Lucia and Moritz Diehl},
doi = {10.1109/LCSYS.2024.3396611},
year = {2024},
date = {2024-03-08},
urldate = {2024-03-08},
journal = {IEEE Control Systems Letters},
volume = {8},
pages = {508-513},
abstract = {We investigate the suboptimality resulting from the application of nominal model predictive control (MPC) to a nonlinear discrete time stochastic system. The suboptimality is defined with respect to the corresponding stochastic optimal control problem (OCP) that minimizes the expected cost of the closed loop system. In this context, nominal MPC corresponds to a form of certainty-equivalent control (CEC). We prove that, in a smooth and unconstrained setting, the suboptimality growth is of fourth order with respect to the level of uncertainty, a parameter which we can think of as a standard deviation. This implies that the suboptimality does not grow very quickly as the level of uncertainty is increased, providing further insight into the practical success of nominal MPC. Similarly, the difference between the optimal and suboptimal control inputs is of second order. We illustrate the result on a simple numerical example, which we also use to show how the proven relationship may cease to hold in the presence of state constraints.},
keywords = {},
pubstate = {published},
tppubtype = {article}
}
Gao, Yunfan; Messerer, Florian; Frey, Jonathan; Duijkeren, Niels; Diehl, Moritz
Collision-free Motion Planning for Mobile Robots by Zero-order Robust Optimization-based MPC Proceedings Article
In: 2023 European Control Conference (ECC), pp. 1-6, IEEE, Bucharest, Romania, 2023, ISBN: 978-3-907144-08-4.
@inproceedings{GaoCFMPECC23,
title = {Collision-free Motion Planning for Mobile Robots by Zero-order Robust Optimization-based MPC},
author = {Yunfan Gao and Florian Messerer and Jonathan Frey and Niels Duijkeren and Moritz Diehl},
url = {https://ieeexplore.ieee.org/document/10178311},
doi = {https://doi.org/10.23919/ECC57647.2023.10178311},
isbn = {978-3-907144-08-4},
year = {2023},
date = {2023-07-17},
urldate = {2023-07-17},
booktitle = {2023 European Control Conference (ECC)},
pages = {1-6},
publisher = {IEEE},
address = {Bucharest, Romania},
abstract = {This paper presents an implementation of robust model predictive control (MPC) for collision-free reference trajectory tracking for mobile robots. The presented approach considers the robot motion to be subject to process noise bounded by ellipsoidal sets. In order to efficiently handle the evolution of the disturbance ellipsoids within the MPC, the zero-order robust optimization (zoRO) scheme is applied [1]. The idea is to fix the disturbance ellipsoids within one optimization iteration and solve the problem repeatedly with updated disturbance ellipsoid trajectories. The zero-order approach is suboptimal in general. However, we show that it does not impair convergence to the reference trajectory in the absence of obstacles. The experiments on an industrial mobile robot prototype demonstrate the performance of the controller.},
keywords = {},
pubstate = {published},
tppubtype = {inproceedings}
}
Baumgärtner, Katrin; Messerer, Florian; Diehl, Moritz
A Unified Local Convergence Analysis of Differential Dynamic Programming, Direct Single Shooting, and Direct Multiple Shooting Proceedings Article
In: 2023 European Control Conference (ECC), pp. 1-7, IEEE, Bucharest, Romania, 2023, ISBN: 978-3-907144-08-4.
@inproceedings{Baumgaertner2023a,
title = {A Unified Local Convergence Analysis of Differential Dynamic Programming, Direct Single Shooting, and Direct Multiple Shooting},
author = {Katrin Baumgärtner and Florian Messerer and Moritz Diehl},
url = {https://ieeexplore.ieee.org/document/10178367},
doi = {https://doi.org/10.23919/ECC57647.2023.10178367},
isbn = {978-3-907144-08-4},
year = {2023},
date = {2023-07-17},
urldate = {2023-07-17},
booktitle = {2023 European Control Conference (ECC)},
pages = {1-7},
publisher = {IEEE},
address = {Bucharest, Romania},
abstract = {We revisit three classical numerical methods for solving unconstrained optimal control problems - differential dynamic programming, direct single shooting, and direct multiple shooting - and examine their local convergence behaviour. In particular, we show that all three methods converge with the same linear rate if a Gauss-Newton (GN) - or Generalized Gauss-Newton (GGN) - Hessian approximation is used, which is the case in widely used implementations such as iLQR.},
keywords = {},
pubstate = {published},
tppubtype = {inproceedings}
}
Ghezzi, Andrea; Messerer, Florian; Balocco, Jacopo; Manzoni, Vincenzo; Diehl, Moritz
An Implicit and Explicit Dual Model Predictive Control Formulation for a Steel Recycling Process Journal Article
In: European Journal of Control, pp. 100841, 2023, ISSN: 0947-3580.
@article{GHEZZI2023100841,
title = {An Implicit and Explicit Dual Model Predictive Control Formulation for a Steel Recycling Process},
author = {Andrea Ghezzi and Florian Messerer and Jacopo Balocco and Vincenzo Manzoni and Moritz Diehl},
url = {https://www.sciencedirect.com/science/article/pii/S0947358023000705},
doi = {https://doi.org/10.1016/j.ejcon.2023.100841},
issn = {0947-3580},
year = {2023},
date = {2023-06-14},
urldate = {2023-06-14},
journal = {European Journal of Control},
pages = {100841},
abstract = {We present a formulation for both implicit and explicit dual model predictive control for a steel recycling process. The process consists in the production of new steel by choosing a combination of several different steel scraps with unknown pollutant content. The pollutant content can only be measured after a scrap combination is molten, allowing for inference on the pollutants in the different scrap heaps. The production cost should be minimized while ensuring high quality of the product through constraining the maximum amount of pollutant. The dual control formulation allows to achieve the optimal explore-exploit trade-off between uncertainty reduction and cost minimization for the examined problem. Specifically, the dual effect is obtained by considering the dependence of the future pollutant uncertainties on the scrap selection in the predictions. The implicit formulation promotes uncertainty reduction indirectly via the impact of active constraints on the objective, while the explicit formulation adds a heuristic cost on uncertainty to encourage active exploration. We compare the formulations by numerical simulations of a simplified but representative industrial steel recycling process. The results demonstrate the superiority of the two dual formulations with respect to a robustified but non-dual formulation.},
keywords = {},
pubstate = {published},
tppubtype = {article}
}
Messerer, Florian; Baumgärtner, Katrin; Diehl, Moritz
A Dual-Control Effect Preserving Formulation for Nonlinear Output-Feedback Stochastic Model Predictive Control With Constraints Journal Article
In: IEEE Control Systems Letters, vol. 7, no. 1171--1176, 2023.
@article{Messerer2023,
title = {A Dual-Control Effect Preserving Formulation for Nonlinear Output-Feedback Stochastic Model Predictive Control With Constraints},
author = {Florian Messerer and Katrin Baumgärtner and Moritz Diehl},
url = {https://ieeexplore.ieee.org/document/9993720},
doi = {10.1109/LCSYS.2022.3230552},
year = {2023},
date = {2023-01-01},
urldate = {2023-01-01},
journal = {IEEE Control Systems Letters},
volume = {7},
number = {1171--1176},
keywords = {},
pubstate = {published},
tppubtype = {article}
}
Reiter, Rudolf; Messerer, Florian; Schratter, Markus; Watzenig, Daniel; Diehl, Moritz
An Inverse Optimal Control Approach for Trajectory Prediction of Autonomous Race Cars Proceedings Article
In: 2022 European Control Conference (ECC), pp. 146–153, IEEE, London, United Kingdom, 2022, ISBN: 978-3-907144-07-7.
@inproceedings{reiter_inverse_2022,
title = {An Inverse Optimal Control Approach for Trajectory Prediction of Autonomous Race Cars},
author = {Rudolf Reiter and Florian Messerer and Markus Schratter and Daniel Watzenig and Moritz Diehl},
url = {https://ieeexplore.ieee.org/document/9838100/},
doi = {10.23919/ECC55457.2022.9838100},
isbn = {978-3-907144-07-7},
year = {2022},
date = {2022-07-01},
urldate = {2022-09-09},
booktitle = {2022 European Control Conference (ECC)},
pages = {146--153},
publisher = {IEEE},
address = {London, United Kingdom},
abstract = {This paper proposes an optimization-based approach to predict trajectories of autonomous race cars. We assume that the observed trajectory is the result of an optimization problem that trades off path progress against acceleration and jerk smoothness, and which is restricted by constraints. The algorithm predicts a trajectory by solving a parameterized nonlinear program (NLP) which contains path progress and smoothness in cost terms. By observing the actual motion of a vehicle, the parameters of prediction are updated by means of solving an inverse optimal control problem that contains the parameters of the predicting NLP as optimization variables. The algorithm therefore learns to predict the observed vehicle trajectory in a least-squares relation to measurement data and to the presumed structure of the predicting NLP. This work contributes with an algorithm that allows for accurate and interpretable predictions with sparse data. The algorithm is implemented on embedded hardware in an autonomous real-world race car that is competing in the challenge Roborace and analyzed with respect to recorded data.},
keywords = {},
pubstate = {published},
tppubtype = {inproceedings}
}