Yunfan Gao
PhD Candidate
Advanced Autonomous Systems Department
Robert Bosch GmbH, Corporate Research
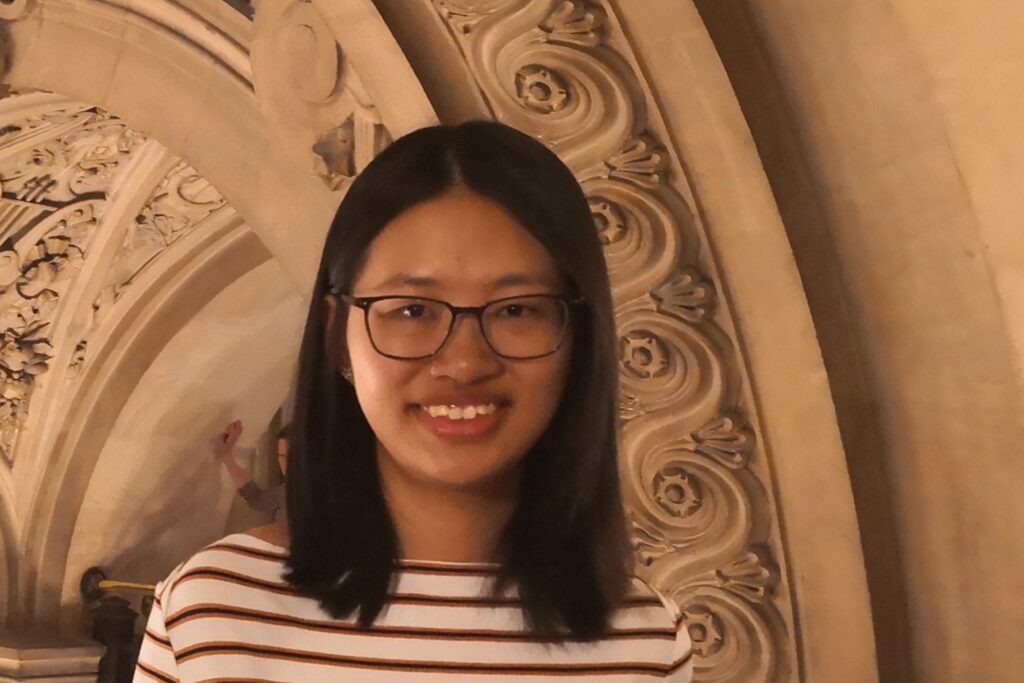
Yunfan Gao obtained the bachelor degree in Electronic engineering from Fudan University, Shanghai, China in 2019. Then she took her master study in Robotics, Systems and Control at ETH Zurich, and graduated in January 2022. Her master thesis was about the integration of projection mapping with mobile robots. Recently in March 2022, she started carrying out a PhD at Bosch Research with the topic “Safety and Robustness in Mobile Robot Motion Planning”.
Project description
This PhD project is about motion planning for industrial robots and service robots. The aim is to run mobile robots efficiently with safety guaranteed. Currently, the common approach in industry to achieve safety is to deploy a separate safety controller. The controller alters the control commands made by the motion planner if necessary. This approach is too conservative as large portions of the space are marked as unsafe. To achieve safety in motion planning non-conservatively is challenging. Possible research directions include tighter integration between the motion planning and the safety control, prediction of surrounding agents’ trajectories, as well as real-time execution.
Gao, Yunfan; Messerer, Florian; van Duijkeren, Niels; Houska, Boris; Diehl, Moritz
Real-Time-Feasible Collision-Free Motion Planning For Ellipsoidal Objects Proceedings Article Forthcoming
In: Forthcoming, (Accepted at the 2024 Conference on Decision and Control (CDC)).
@inproceedings{24_gao_realtimefeasible,
title = {Real-Time-Feasible Collision-Free Motion Planning For Ellipsoidal Objects},
author = {Yunfan Gao and Florian Messerer and Niels van Duijkeren and Boris Houska and Moritz Diehl},
doi = {https://doi.org/10.48550/arXiv.2409.12007},
year = {2024},
date = {2024-09-18},
abstract = {Online planning of collision-free trajectories is a fundamental task for robotics and self-driving car applications. This paper revisits collision avoidance between ellipsoidal objects using differentiable constraints. Two ellipsoids do not overlap if and only if the endpoint of the vector between the center points of the ellipsoids does not lie in the interior of the Minkowski sum of the ellipsoids. This condition is formulated using a parametric over-approximation of the Minkowski sum, which can be made tight in any given direction. The resulting collision avoidance constraint is included in an optimal control problem (OCP) and evaluated in comparison to the separating-hyperplane approach. Not only do we observe that the Minkowski-sum formulation is computationally more efficient in our experiments, but also that using pre-determined over-approximation parameters based on warm-start trajectories leads to a very limited increase in suboptimality. This gives rise to a novel real-time scheme for collision-free motion planning with model predictive control (MPC). Both the real-time feasibility and the effectiveness of the constraint formulation are demonstrated in challenging real-world experiments.},
note = {Accepted at the 2024 Conference on Decision and Control (CDC)},
keywords = {},
pubstate = {forthcoming},
tppubtype = {inproceedings}
}
Gao, Yunfan; Messerer, Florian; van Duijkeren, Niels; Diehl, Moritz
Stochastic Model Predictive Control with Optimal Linear Feedback for Mobile Robots in Dynamic Environments Proceedings Article
In: 8th IFAC Conference on Nonlinear Model Predictive Control NMPC 2024, pp. 153-158, IFAC-PapersOnLine, 2024.
@inproceedings{24_gao_stochasticmpc,
title = {Stochastic Model Predictive Control with Optimal Linear Feedback for Mobile Robots in Dynamic Environments},
author = {Yunfan Gao and Florian Messerer and Niels van Duijkeren and Moritz Diehl},
doi = {https://doi.org/10.1016/j.ifacol.2024.09.024},
year = {2024},
date = {2024-09-03},
urldate = {2024-09-03},
booktitle = {8th IFAC Conference on Nonlinear Model Predictive Control NMPC 2024},
volume = {58},
number = {18},
pages = {153-158},
publisher = {IFAC-PapersOnLine},
abstract = {Robot navigation around humans can be a challenging problem since human movements are hard to predict. Stochastic model predictive control (MPC) can account for such uncertainties and approximately bound the probability of a collision to take place. In this paper, to counteract the rapidly growing human motion uncertainty over time, we incorporate state feedback in the stochastic MPC. This allows the robot to more closely track reference trajectories. To this end the feedback policy is left as a degree of freedom in the optimal control problem. The stochastic MPC with feedback is validated in simulation experiments and is compared against nominal MPC and stochastic MPC without feedback. The added computation time can be limited by reducing the number of additional variables for the feedback law with a small compromise in control performance.},
keywords = {},
pubstate = {published},
tppubtype = {inproceedings}
}
Frey, Jonathan; Gao, Yunfan; Messerer, Florian; Lahr, Amon; Zeilinger, Melanie N.; Diehl, Moritz
Efficient Zero-Order Robust Optimization for Real-Time Model Predictive Control with Acados Proceedings Article
In: 2024 European Control Conference (ECC), IEEE, Stockholm, Sweden, 2024, ISBN: 978-3-9071-4410-7.
@inproceedings{frey_efficient_2023,
title = {Efficient Zero-Order Robust Optimization for Real-Time Model Predictive Control with Acados},
author = {Jonathan Frey and Yunfan Gao and Florian Messerer and Amon Lahr and Melanie N. Zeilinger and Moritz Diehl},
doi = {10.23919/ECC64448.2024.10591148},
isbn = {978-3-9071-4410-7},
year = {2024},
date = {2024-06-03},
urldate = {2023-12-18},
booktitle = {2024 European Control Conference (ECC)},
publisher = {IEEE},
address = {Stockholm, Sweden},
abstract = {Robust and stochastic optimal control problem (OCP) formulations allow a systematic treatment of uncertainty, but are typically associated with a high computational cost. The recently proposed zero-order robust optimization (zoRO) algorithm mitigates the computational cost of uncertainty-aware MPC by propagating the uncertainties separately from the nominal dynamics. This paper details the combination of zoRO with the real-time iteration (RTI) scheme and presents an efficient open-source implementation in acados, utilizing BLASFEO for the linear algebra operations. In addition to the scaling advantages posed by the zoRO algorithm, the efficient implementation drastically reduces the computational overhead, and, combined with an RTI scheme, enables the use of tube-based MPC for a wider range of applications. The flexibility, usability and effectiveness of the proposed implementation is demonstrated on two examples. On the practical example of a differential drive robot, the proposed implementation results in a tenfold reduction of computation time with respect to the previously available zoRO implementation.},
keywords = {},
pubstate = {published},
tppubtype = {inproceedings}
}
Gao, Yunfan; Messerer, Florian; Frey, Jonathan; Duijkeren, Niels; Diehl, Moritz
Collision-free Motion Planning for Mobile Robots by Zero-order Robust Optimization-based MPC Proceedings Article
In: 2023 European Control Conference (ECC), pp. 1-6, IEEE, Bucharest, Romania, 2023, ISBN: 978-3-907144-08-4.
@inproceedings{GaoCFMPECC23,
title = {Collision-free Motion Planning for Mobile Robots by Zero-order Robust Optimization-based MPC},
author = {Yunfan Gao and Florian Messerer and Jonathan Frey and Niels Duijkeren and Moritz Diehl},
url = {https://ieeexplore.ieee.org/document/10178311},
doi = {https://doi.org/10.23919/ECC57647.2023.10178311},
isbn = {978-3-907144-08-4},
year = {2023},
date = {2023-07-17},
urldate = {2023-07-17},
booktitle = {2023 European Control Conference (ECC)},
pages = {1-6},
publisher = {IEEE},
address = {Bucharest, Romania},
abstract = {This paper presents an implementation of robust model predictive control (MPC) for collision-free reference trajectory tracking for mobile robots. The presented approach considers the robot motion to be subject to process noise bounded by ellipsoidal sets. In order to efficiently handle the evolution of the disturbance ellipsoids within the MPC, the zero-order robust optimization (zoRO) scheme is applied [1]. The idea is to fix the disturbance ellipsoids within one optimization iteration and solve the problem repeatedly with updated disturbance ellipsoid trajectories. The zero-order approach is suboptimal in general. However, we show that it does not impair convergence to the reference trajectory in the absence of obstacles. The experiments on an industrial mobile robot prototype demonstrate the performance of the controller.},
keywords = {},
pubstate = {published},
tppubtype = {inproceedings}
}