Katrin Baumgärtner
PhD Candidate in Engineering Science
University of Freiburg
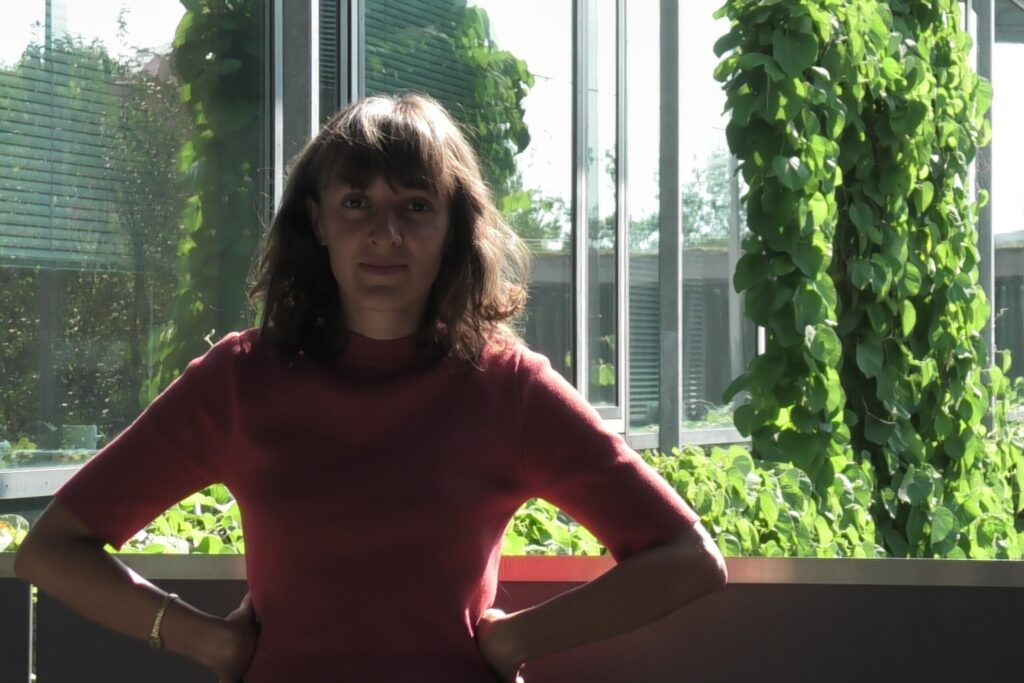
Katrin Baumgärtner was born in Ulm, Germany, in 1993. She obtained her Master degree in Computer Science from the University of Freiburg with a thesis on Asymptotic Comparison of Bayesian State Estimates and Numerical Methods for Moving Horizon Estimation. From November 2019 she joined the SYSCOP Laboratory at the University of Freiburg, carrying out a PhD under the supervision of Prof. Dr. Moritz Diehl.
Project description
For dynamic processes where only approximate models are available, data-driven strategies that adapt the model and/or the control problem formulation online need to be employed in order to ensure optimal control performance. On the one hand, the system model might include white-box parameters, i.e. some constants within a physical model, that are not known exactly or might change over the course of operation. On the other hand, if the system exhibits behaviour for which no exact model is known or the available models are too computationally demanding to be used within a feedback control system, one might introduce additional black-box parameters. These parameters do not have a physical interpretation, but allow for a model correction in order to (locally or globally) explain the observed system behaviour. The focus of my PhD is to develop optimal control and estimation problem formulations as well as numerical algorithms to efficiently identify unknown white-box parameters as well as black-box model corrections from data leading to adaptive optimal control strategies.
For estimation of white-box parameters, Moving Horizon Estimation (MHE) is a powerful framework that allows us to explicitly account for various noise distributions and additional nonlinear constraints. The main challenge for applying MHE in practice is its computational complexity. As part of my PhD,
I have been exploring tailored algorithms that exploit the particular structure of the MHE problem or use approximate formulations. Future research will include formulations with non-Gaussian noise distributions leading to non-convex loss functions for which specialized numerical optimization
methods can be leveraged.
If models with black-box components are used, it can be advantageous to explicitly take into account the control problem formulation to identify those parts of the state space where the cost function is highly sensitive to modeling errors and, maybe even more importantly, where it is not affected
by small changes of the model equations. Future research will include algorithms for active system identification that exploit the structure of the optimal control problem at hand when selecting those directions in the state space in which the system behaviour is to be explored.
Publications
Messerer, Florian; Baumgärtner, Katrin; Nurkanovic, Armin; Diehl, Moritz
Approximate propagation of normal distributions for stochastic optimal control of nonsmooth systems Journal Article
In: Nonlinear Analysis: Hybrid Systems, vol. 53, pp. 101499, 2024, ISSN: 1751-570X.
@article{MESSERER2024101499,
title = {Approximate propagation of normal distributions for stochastic optimal control of nonsmooth systems},
author = {Florian Messerer and Katrin Baumgärtner and Armin Nurkanovic and Moritz Diehl},
url = {https://www.sciencedirect.com/science/article/pii/S1751570X24000360},
doi = {https://doi.org/10.1016/j.nahs.2024.101499},
issn = {1751-570X},
year = {2024},
date = {2024-08-01},
urldate = {2023-08-14},
journal = {Nonlinear Analysis: Hybrid Systems},
volume = {53},
pages = {101499},
abstract = {We present a method for the approximate propagation of mean and covariance of a probability distribution through ordinary differential equations (ODE) with discontinuous right-hand side. For piecewise affine systems, a normalization of the propagated probability distribution at every time step allows us to analytically compute the expectation integrals of the mean and covariance dynamics while explicitly taking into account the discontinuity. This leads to a natural smoothing of the discontinuity such that for relevant levels of uncertainty the resulting ODE can be integrated directly with standard schemes and it is neither necessary to prespecify the switching sequence nor to use a switch detection method. We then show how this result can be employed in the more general case of piecewise smooth functions based on a structure preserving linearization scheme. The resulting dynamics can be straightforwardly used within standard formulations of stochastic optimal control problems with chance constraints.},
keywords = {},
pubstate = {published},
tppubtype = {article}
}
Messerer, Florian; Baumgärtner, Katrin; Lucia, Sergio; Diehl, Moritz
Fourth-order suboptimality of nominal model predictive control in the presence of uncertainty Journal Article
In: IEEE Control Systems Letters, vol. 8, pp. 508-513, 2024.
@article{messerer2024fourthorder,
title = {Fourth-order suboptimality of nominal model predictive control in the presence of uncertainty},
author = {Florian Messerer and Katrin Baumgärtner and Sergio Lucia and Moritz Diehl},
doi = {10.1109/LCSYS.2024.3396611},
year = {2024},
date = {2024-03-08},
urldate = {2024-03-08},
journal = {IEEE Control Systems Letters},
volume = {8},
pages = {508-513},
abstract = {We investigate the suboptimality resulting from the application of nominal model predictive control (MPC) to a nonlinear discrete time stochastic system. The suboptimality is defined with respect to the corresponding stochastic optimal control problem (OCP) that minimizes the expected cost of the closed loop system. In this context, nominal MPC corresponds to a form of certainty-equivalent control (CEC). We prove that, in a smooth and unconstrained setting, the suboptimality growth is of fourth order with respect to the level of uncertainty, a parameter which we can think of as a standard deviation. This implies that the suboptimality does not grow very quickly as the level of uncertainty is increased, providing further insight into the practical success of nominal MPC. Similarly, the difference between the optimal and suboptimal control inputs is of second order. We illustrate the result on a simple numerical example, which we also use to show how the proven relationship may cease to hold in the presence of state constraints.},
keywords = {},
pubstate = {published},
tppubtype = {article}
}
Baumgärtner, Katrin; Diehl, Moritz
Local Convergence Analysis of Damping for Zero-Order Optimization-Based Iterative Learning Control Proceedings Article
In: 2023 European Control Conference (ECC), pp. 1-6, IEEE, Bucharest, Romania, 2023, ISBN: 978-3-907144-08-4.
@inproceedings{Baumgaertner2023,
title = {Local Convergence Analysis of Damping for Zero-Order Optimization-Based Iterative Learning Control},
author = {Katrin Baumgärtner and Moritz Diehl},
url = {https://ieeexplore.ieee.org/document/10178225},
doi = {https://doi.org/10.23919/ECC57647.2023.10178225},
isbn = {978-3-907144-08-4},
year = {2023},
date = {2023-07-17},
urldate = {2023-07-17},
booktitle = {2023 European Control Conference (ECC)},
pages = {1-6},
publisher = {IEEE},
address = {Bucharest, Romania},
abstract = {Within the Iterative Learning Control (ILC) framework, damping is often introduced as a heuristic to facilitate convergence of the ILC iterates. We analyze how two simple damping approaches affect the local convergence behaviour of a zero-order optimization-based ILC method introduced in [1] and prove that the condition for local convergence, which is given in terms of the eigenvalues of an iteration matrix, can be relaxed if damping is introduced. Leveraging a simple example, we illustrate the effects of damping, which might be (1) convergence of an initially diverging iteration or (2) acceleration or deceleration of a converging iteration.},
keywords = {},
pubstate = {published},
tppubtype = {inproceedings}
}
Baumgärtner, Katrin; Messerer, Florian; Diehl, Moritz
A Unified Local Convergence Analysis of Differential Dynamic Programming, Direct Single Shooting, and Direct Multiple Shooting Proceedings Article
In: 2023 European Control Conference (ECC), pp. 1-7, IEEE, Bucharest, Romania, 2023, ISBN: 978-3-907144-08-4.
@inproceedings{Baumgaertner2023a,
title = {A Unified Local Convergence Analysis of Differential Dynamic Programming, Direct Single Shooting, and Direct Multiple Shooting},
author = {Katrin Baumgärtner and Florian Messerer and Moritz Diehl},
url = {https://ieeexplore.ieee.org/document/10178367},
doi = {https://doi.org/10.23919/ECC57647.2023.10178367},
isbn = {978-3-907144-08-4},
year = {2023},
date = {2023-07-17},
urldate = {2023-07-17},
booktitle = {2023 European Control Conference (ECC)},
pages = {1-7},
publisher = {IEEE},
address = {Bucharest, Romania},
abstract = {We revisit three classical numerical methods for solving unconstrained optimal control problems - differential dynamic programming, direct single shooting, and direct multiple shooting - and examine their local convergence behaviour. In particular, we show that all three methods converge with the same linear rate if a Gauss-Newton (GN) - or Generalized Gauss-Newton (GGN) - Hessian approximation is used, which is the case in widely used implementations such as iLQR.},
keywords = {},
pubstate = {published},
tppubtype = {inproceedings}
}
Baumgärtner, Katrin; Zanelli, Andrea; Diehl, Moritz
Stability Analysis of Nonlinear Model Predictive Control with Progressive Tightening of Stage Costs and Constraints Journal Article
In: IEEE Control Systems Letters, vol. 7, pp. 3018-3023, 2023, ISSN: 2475-1456.
@article{Baumgaertner2023b,
title = {Stability Analysis of Nonlinear Model Predictive Control with Progressive Tightening of Stage Costs and Constraints},
author = {Katrin Baumgärtner and Andrea Zanelli and Moritz Diehl},
doi = {https://doi.org/10.1109/LCSYS.2023.3289707},
issn = {2475-1456},
year = {2023},
date = {2023-06-26},
journal = {IEEE Control Systems Letters},
volume = {7},
pages = {3018-3023},
abstract = {We consider a stage-varying nonlinear model predictive control (NMPC) formulation and provide a stability result for the corresponding closed-loop system under the assumption that cost and constraints are progressively tightening. We illustrate the generality of the stage-varying formulation pointing out various approaches proposed in the literature that can be cast as stage-varying and progressively tightening optimal control problems.},
keywords = {},
pubstate = {published},
tppubtype = {article}
}
Baumgärtner, Katrin; Wang, Yizhen; Zanelli, Andrea; Diehl, Moritz
Fast Nonlinear Model Predictive Control using Barrier Formulations and Squashing with a Generalized Gauss-Newton Hessian Proceedings Article
In: 2022 IEEE 61st Conference on Decision and Control (CDC), pp. 558-563, IEEE, 2023, ISBN: 978-1-6654-6761-2.
@inproceedings{Baumgaertner2022a,
title = {Fast Nonlinear Model Predictive Control using Barrier Formulations and Squashing with a Generalized Gauss-Newton Hessian},
author = {Katrin Baumgärtner and Yizhen Wang and Andrea Zanelli and Moritz Diehl},
url = {https://ieeexplore.ieee.org/document/9992869},
doi = {https://doi.org/10.1109/CDC51059.2022.9992869},
isbn = {978-1-6654-6761-2},
year = {2023},
date = {2023-01-10},
urldate = {2023-01-10},
booktitle = {2022 IEEE 61st Conference on Decision and Control (CDC)},
pages = {558-563},
publisher = {IEEE},
abstract = {We propose an approximate algorithm for Nonlinear Model Predictive Control (NMPC) which is based on a reformulation of the inequality constrained optimal control problem using barrier terms and squashing functions. Within an SQP framework, the particular structure of the reformulated problem can be leveraged by using a Generalized Gauss-Newton Hessian approximation. Moreover, the quadratic subproblems can be efficiently solved using a single backward and forward sweep of the Riccati recursion. We show local linear convergence of the proposed algorithm, as well as local quadratic convergence in the case of linear system dynamics. The computational speed-up, which can be achieved with the proposed method, is illustrated in a simulation study.},
keywords = {},
pubstate = {published},
tppubtype = {inproceedings}
}
Baumgärtner, Katrin; Reiter, Rudolf; Diehl, Moritz
Moving Horizon Estimation with Adaptive Regularization for Ill-Posed State and Parameter Estimation Problems Proceedings Article
In: 2022 IEEE 61st Conference on Decision and Control (CDC), pp. 2165-2171, IEEE, Cancun, Mexico, 2023, ISBN: 978-1-6654-6761-2.
@inproceedings{Baumgärtner2022MHE,
title = {Moving Horizon Estimation with Adaptive Regularization for Ill-Posed State and Parameter Estimation Problems},
author = {Katrin Baumgärtner and Rudolf Reiter and Moritz Diehl},
doi = {10.1109/CDC51059.2022.9993416},
isbn = {978-1-6654-6761-2},
year = {2023},
date = {2023-01-10},
urldate = {2023-01-10},
booktitle = {2022 IEEE 61st Conference on Decision and Control (CDC)},
pages = {2165-2171},
publisher = {IEEE},
address = {Cancun, Mexico},
abstract = {We investigate the usage of Moving Horizon Estimation (MHE) for state and parameter estimation for partially non-detectable systems with measurements corrupted by outliers. We propose an arrival cost update formula based on the Generalized Gauss-Newton method and illustrate how it can be generalized to nonconvex loss functions that can be effectively used for outlier rejection. Moreover, we propose an adaptive regularization scheme for the arrival cost which introduces forgetting as well as additional pseudo-measurements to the arrival cost update. We illustrate the performance of the proposed algorithms on a longitudinal vehicle state and parameter estimation problem.},
keywords = {},
pubstate = {published},
tppubtype = {inproceedings}
}
Messerer, Florian; Baumgärtner, Katrin; Diehl, Moritz
A Dual-Control Effect Preserving Formulation for Nonlinear Output-Feedback Stochastic Model Predictive Control With Constraints Journal Article
In: IEEE Control Systems Letters, vol. 7, no. 1171--1176, 2023.
@article{Messerer2023,
title = {A Dual-Control Effect Preserving Formulation for Nonlinear Output-Feedback Stochastic Model Predictive Control With Constraints},
author = {Florian Messerer and Katrin Baumgärtner and Moritz Diehl},
url = {https://ieeexplore.ieee.org/document/9993720},
doi = {10.1109/LCSYS.2022.3230552},
year = {2023},
date = {2023-01-01},
urldate = {2023-01-01},
journal = {IEEE Control Systems Letters},
volume = {7},
number = {1171--1176},
keywords = {},
pubstate = {published},
tppubtype = {article}
}
Cecchin, Leonardo; Baumgärtner, Katrin; Gering, Stefan; Diehl, Moritz
Locally Weighted Regression with Approximate Derivatives for Data-based optimization Proceedings Article
In: 2023 European Control Conference (ECC), pp. 1–6, IEEE 2023.
@inproceedings{cecchin2023locally,
title = {Locally Weighted Regression with Approximate Derivatives for Data-based optimization},
author = { Leonardo Cecchin and Katrin Baumgärtner and Stefan Gering and Moritz Diehl},
year = {2023},
date = {2023-01-01},
urldate = {2023-01-01},
booktitle = {2023 European Control Conference (ECC)},
pages = {1–6},
organization = {IEEE},
abstract = {Interpolation and approximation of data provided in terms of a Look-Up Table (LUT) is a common and well-known task, and is especially relevant for industrial applications. When using the function for point-wise evaluation, the method choice only affects the accuracy of the function value itself. However, when the LUT is used as part of an optimization problem formulation, a bad method choice can prevent convergence or alter significantly the outcome of the solver. Moreover, computational efficiency becomes critical due to the much higher number of evaluations required. This work focuses on a variation of Locally Weighted Regression, with approximate derivatives computation. The result is a method that allows one to obtain the function value together with the first n derivatives, at a reduced computational cost. Theoretical properties of the approach are analyzed, and the results of a minimization problem using the proposed method are compared with more traditional ones. The new approach shows promising performance and results, both for computational efficiency and effectiveness when used in optimization.},
keywords = {},
pubstate = {published},
tppubtype = {inproceedings}
}
Baumgärtner, Katrin; Diehl, Moritz
The Extended Gauss-Newton Method for Nonconvex Loss Functions and its Application to Time-Optimal Model Predictive Control Proceedings Article
In: 2022 American Control Conference (ACC), pp. 4973-4978, IEEE, 2022, ISBN: 978-1-6654-5196-3.
@inproceedings{Baumgaertner2022,
title = {The Extended Gauss-Newton Method for Nonconvex Loss Functions and its Application to Time-Optimal Model Predictive Control},
author = {Katrin Baumgärtner and Moritz Diehl},
url = {https://ieeexplore.ieee.org/document/9867372},
doi = {https://doi.org/10.23919/ACC53348.2022.9867372},
isbn = {978-1-6654-5196-3},
year = {2022},
date = {2022-09-05},
urldate = {2022-09-05},
booktitle = {2022 American Control Conference (ACC)},
pages = {4973-4978},
publisher = {IEEE},
abstract = {We introduce the eXtended Gauss-Newton (XGN) method, which extends the Generalized Gauss-Newton (GGN) method to a class of nonconvex loss functions with a well-behaved global minimum. We show linear local convergence of the XGN method to a stationary point, as well as global convergence in case of a linear residual function and linear constraints. As one possible application, we illustrate how the considered class of nonconvex loss functions can be used to approximate time-optimal behaviour within a Model Predictive Control (MPC) framework.},
keywords = {},
pubstate = {published},
tppubtype = {inproceedings}
}